Keynote speakers
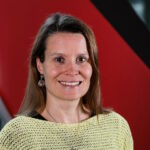
Tanja Käser
EPFL School of Computer and Communication Sciences
Tanja Käser is an assistant professor at the EPFL School of Computer and Communication Sciences (IC) and head of the Machine Learning for Education laboratory. Her research lies at the intersection of machine learning, data mining, and education. She is particularly interested in creating accurate models of human behavior and learning. Prior to joining EPFL, Tanja Käser was a senior data scientist with the Swiss Data Science Center at ETH Zurich. Before that, she was a postdoctoral researcher with the AAALab at the Graduate School of Education of Stanford University. Tanja Käser received her PhD degree from the Computer Science Department of ETH Zurich; her thesis was distinguished with the Fritz Kutter Award for the best Computer Science thesis at a Swiss university.
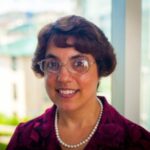
Carolyn Rosé
Professor of Language Technologies and
Human-Computer Interaction
School of Computer Science, Carnegie
Mellon, USA
Dr. Carolyn Rosé is a Professor of Language Technologies and
Human-Computer Interaction in the School of Computer Science at Carnegie
Mellon, and Program Director for the Masters of Computational Data
Science Program. Her research program focuses on computational modeling
of discourse to enable scientific understanding the social and pragmatic
nature of conversational interaction of all forms, and using this
understanding to build intelligent computational systems for improving
collaborative interactions. She is best known for her work on dynamic
support of collaborative learning using intelligent conversational
agents in online, face-to-face, and hybrid settings, triggered through
real time analysis of conversational interactions. Her research group’s
highly interdisciplinary work, published in well over 300 peer reviewed
publications, is represented in the top venues of 5 fields: namely,
Language Technologies, Learning Sciences, Cognitive Science, Educational
Technology, and Human-Computer Interaction, with awards in 4 of these
fields. She is a Past President and Inaugural Fellow of the
International Society of the Learning Sciences, Senior member of IEEE,
Founding Chair of the International Alliance to Advance Learning in the
Digital Era, and Executive Editor (formerly Co-Editor-in-Chief) of the
International Journal of Computer-Supported Collaborative Learning. She
also serves as a 2020-2021 AAAS Leshner Leadership Institute Fellow for
Public Engagement with Science, with a focus on public engagement with
Artificial Intelligence.
Test of Time Award Talk
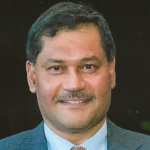
Gautam Biswas
Professor of Computer Science, Vanderbilt University, Nashville, TN. USA.
Bio. Gautam Biswas conducts research in AI and Machine Learning with primary interests in developing intelligent open-ended learning environments focused on learning and instruction in STEM + CS domains. He has developed innovative learning analytics and data mining techniques for studying students’ learning behaviors and linking them to their metacognitive and self-regulated learning strategies. More recently, he has been analyzing multi-modal data from augmented reality training environments to study individual and team performance. Prof. Biswas also conducts research in monitoring, control, and fault adaptivity of complex cyber-physical systems. In particular, his research focuses on Deep Reinforcement Learning, Unsupervised and Semi-supervised Anomaly Detection methods, and Online Risk and Safety analysis applied to Air and Marine vehicles as well as Smart Buildings. His research has been supported by funding from the Army, Navy, NASA, NSF, DARPA, and the US Department of Education. He has published extensively and currently has over 600-refereed publications. Currently, he is a co-PI of an NSF AI Institute for Engaged Learning (ENGAGE AI)
Title: Assessing Student Learning in Open Ended Learning Environments From Sequential to Multimodal Data Analysis
Abstract. From my early days as an AIED and EDM researcher, I have focused on understanding how students learn, especially in scenarios where they have to construct and apply their knowledge to problem-solving tasks. Collaborating with peers, we developed open-ended learning environments (OELEs) where K-12 students build scientific models and apply them to solve real-world problems. Challenges arise, as students have to navigate with multiple tools in the computer-environments. Some students overcome these challenges to become effective learners while others struggle to progress often applying suboptimal learning strategies. John Kinnebrew and I began analyzing learners’ activity logs to study these differences, resulting in the Differential Sequence Mining algorithm, which earned us the best paper award at EDM 2012. Expanding on this work, we developed the Contextualized Difference Mining method for understanding students’ learning behaviors, for which we are receiving this Test of Time award.
In my talk, I will review our work on Differential sequence mining, and explore its applications in understanding students’ cognitive and metacognitive learning behaviors. We have leveraged these insights to provide adaptive support, helping students’ progress in our Open-Ended Learning Environments (OELEs). Beyond this, we have employed other sequential representations, such as Markov Chains and Hidden Markov Models, to analyze students’ activities and behaviors in the context of their learning and problem-solving tasks. Beyond this, our OELEs have advanced to facilitate students’ integrated learning of science, computing, and engineering problem-solving, including collaborative efforts in computer-based and embodied learning scenarios. From these richer learning environments, I will share insights into our latest efforts involving multimodal data analysis, incorporating video, speech, and activity logs. Using vision-based deep learning models and large language models (LLMs), we integrate analyses across modalities, offering a comprehensive understanding of students’ collaborative learning and problem-solving activities. In conclusion, I will discuss the potential implications of our work on shaping future of learning in classrooms.
EDM data set award
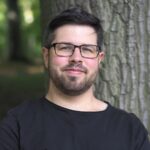
Jakub Kužílek
Senior Researcher, Computer Science Education / Computer Science and Society research group, Humboldt-Universität zu Berlin & German Research Center for Artificial Intelligence
Berlin, Germany.
Bio. Jakub Kužílek is affiliated with the Computer Science Education / Computer Science and Society research group at Humboldt-Universität zu Berlin and the Educational Technology Lab at the German Research Center for Artificial Intelligence (DFKI) as a senior researcher. His research investigates student self-regulated learning within online learning environments, collaborative group work, adaptive assessments, and feedback within the context of digital education. In the past, he developed (together with Martin Hlosta and Zdenek Zdrahal) an OU Analyse system used to support 200.000 students of the Open University (United Kingdom) during their studies and founded learning analytics research at Czech Technical University. He has led (and currently is doing research within) a project on AI use in assessment feedback at Humbold-Universität. In parallel, he is leading the project on AI-driven recommendation systems in vocational education (KIPerWeb at DFKI).
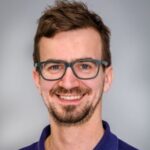
Martin Hlosta
Senior Researcher, Institute for Distance Learning and eLearning Research (IFeL), Swiss distance university of applied sciences (FFHS)
Brig, Switzerland.
Bio. Martin Hlosta is a Senior Researcher at the Institute for Distance Learning and eLearning Research (IFeL) at Swiss distance university of applied sciences (FFHS). Before joining FFHS, he led research and development of OUAnalyse at The Open University (OU) – a Predictive Learning Analytics project deployed in all undergraduate courses, improving student retention and teachers practice. It is one of the world-largest deployment of analytics systems in education and in 2020 it was selected by UNESCO as one of the four best projects using AI in education. His following work focused on identifying factors contributing to large gaps of disadvantaged students in the UK, and in another study presented how using predictive analytics by teachers in an online course can lower these gaps for students coming from low Socio-Economic areas. Currently, he is leading research and teaching in Learning Analytics at FFHS and works on various strands how learning analytics can improve feedback. His most recent project funded by Unity and Meta to target inequalities in education explores how immersive Virtual Reality and enhanced analytics for reflection can help future teachers in South Africa.