ABSTRACT
Studies have shown that on-demand assistance, additional instruction given on a problem per student request, improves student learning in online learning environments. Students may have opinions on whether an assistance was effective at improving student learning. As students are the driving force behind the effectiveness of assistance, there could exist a correlation between students’ perceptions of effectiveness and the computed effectiveness of the assistance. This work conducts a survey asking secondary education students on whether a given assistance is effective in solving a problem in an online learning platform. It then provides a cursory glance at the data to view whether a correlation exists between student perception and the measured effectiveness of an assistance. Over a three year period, approximately twenty-two thousand responses were collected across nearly four thousand, four hundred students. Initial analyses of the survey suggest no significance in the relationship between student perception and computed effectiveness of an assistance, regardless of if the student participated in the survey. All data and analysis conducted can be found on the Open Science Foundation website1.
Keywords
1. INTRODUCTION
Collecting student perceptions on educators’ performances, practices, and generated content has been well established in higher education, along with its ability to potentially improve student instruction [3, 10]. However, very few have been conducted on student perception in secondary education settings [11]. Additionally, surveys conducted on student perception rarely consider individual pieces of content and their effectiveness in context.
On-demand assistance generally improves student leaning [8, 12, 17, 20] in online learning platforms. Educators and their assistance are evaluated through computations to maintain or improve the level of quality and effectiveness [13, 16]. As such, student perceptions on assistance effectiveness are rarely polled, leaving a gap in their evaluation.
In 2017, ASSISTments, an online learning platform [9], deployed the Special Content System, formerly known as TeacherASSIST. The Special Content System allows educators to create and provide on-demand assistance for problems assigned to their students. On-demand assistance was known as student-supports within the application, with most as either hints or explanations. Additionally, educators marked as star-educators had their student-supports provided to any student, regardless of their class, for any problem a class’s educator did not generate a student-support for. The effectiveness of a student-support was evaluated using whether the student answered the next problem in the problem set correctly on their first try [13, 16].
In the Fall semester of 2018, the Special Content System, on providing student-supports to the students, would prompt the student to provide feedback on the helpfulness of the student-supports as shown in Figure 1. The student may have chosen to evaluate the student-support by clicking the "Yes" or "No" button or not responded by completing and moving to the next problem. The feature was disabled in 2020 within the new, redesigned ASSISTments platform; however, educators who have not migrated from the original platform will still be provided this feature: prompting students on the helpfulness of a given student-support.
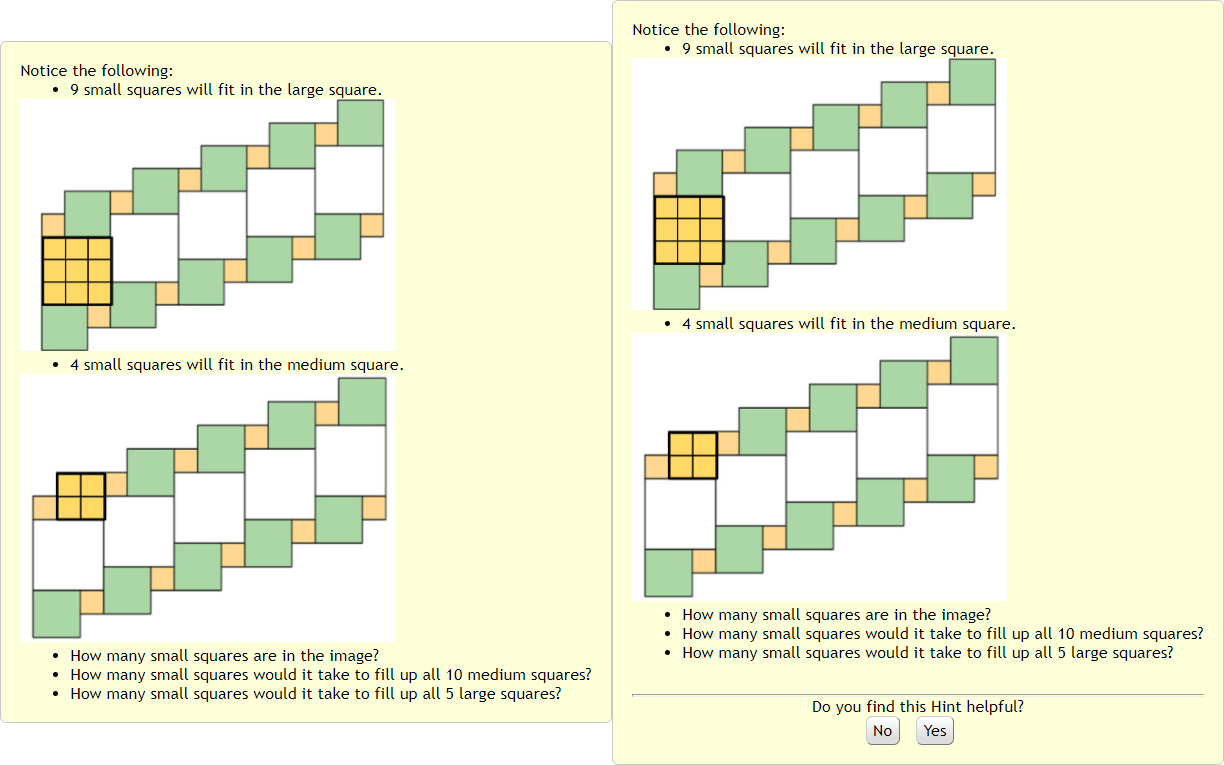
The first part of this work will analyze the students’ perceptions of helpfulness and compare them to the overall effectiveness of the student-support. If correlated, students could be effective in filtering student-supports. In online learning environments which crowdsource their on-demand assistance [13, 12, 17], it may improve automation and independence from moderating bodies. The second part of this work will analyze the students’ perceptions of helpfulness and compare them to the responding students’ effectiveness of the student-support. This may give stronger claims towards a student’s ability to predict the effectiveness of assistance provided to themselves.
In summary, this work aims to answer the following research questions:
- Can student perceptions accurately predict the overall effectiveness of student-supports?
- Can student perceptions accurately predict the effectiveness of student-supports for students who participated in the survey?
2. BACKGROUND
In this work, ASSISTments will be used to conduct the studies. ASSISTments2 is a free, online learning platform providing feedback to inform educators on classroom instruction [9]. ASSISTments provides open source curricula, the majority of which is K-12 mathematics, containing problems and assignments that teachers can assign to their students. Students complete assigned problems in the ASSISTments Tutor. For nearly all problem types, students receive immediate feedback on response submission, which tells the student the correctness of the answer [6]. When a student-support has been written for a problem, a student can request to receive a student-support during the span of problem completion. Student-supports may come in the form of hints which explain how to solve parts of the problems [8, 17], similar problem examples [12], erroneous examples[17, 1], and full solutions to the problems [18, 19].
ASSISTments runs an application known as the Special Content System in which student-supports, usually as hints or explanations, are crowdsourced from educators. Student-supports are created by educators and then provided to students when solving. In this work, data from the ASSISTments Dataset generated by the Special Content System [14, 15] alongside the student perception on student-support effectiveness is used to analyze whether student perceptions accurately predict the effectiveness of student-supports.
3. METHODOLOGY
Data was collected within the original ASSISTments platform over the course of three years. During this time period, when a student-support was provided to a student, a prompt may appear asking about the effectiveness of the student-support, as shown in Figure 1. The student additionally had an equally likely chance that a prompt may not be shown. 46,620 responses across 13,509 students were recorded on the effectiveness of a student-support. Preprocessing on the responses removed any where the effectiveness of a student-support could not be determined. Additionally, any students who only answered one of the available choices across many problems were discarded as the provided answers may not be in full consideration of the question or without major bias. After processing, 21,736 responses across 4,374 students remained. The responses consisted of 5,861 student-supports across 4,120 problems.
After the response were processed, they were grouped by problem and sub-grouped further by student-support. Each sub-group of student-supports contained the data for the student perception of effectiveness and the effectiveness data as specified by the research questions: overall effectiveness and the effectiveness across the students who responded respectively. For every pair of student-supports with a problem group, a Sign Test[5] was performed between the student perception of effectiveness and measured effectiveness of the student-supports. As A Sign Tests measures whether the differences between pair of data is consistent, the results are expected to follow a binomial distribution. As such, a two-sided Binomial Test[4] was performed to measure whether the student perception of effectiveness and the measured effectiveness is consistent. If there was less than two student-supports available for a problem or the difference between the perception or measured effectiveness was zero, then no useful comparison could be made and was thus discarded[5]. The results of the pairwise comparisons between all student-supports are reported using the Pair prefix within Table 1. To make claims on the effectiveness of student-supports on a given problem, another two-sided Binomial Test was performed only on the two supports with the largest combined sample size. The results of the pairwise comparison between the two student-supports with the largest sample size per problem are reported using the Problem prefix within Table 1. As multiple analyses were being performed on the dataset, the Benjamini-Hochberg Procedure[2] was applied to the corresponding p-values to reduce the false discovery rate.
To measure the effectiveness across only those who participated in the survey, a Chi-squared Test[7] was performed comparing the relationship between the student perception of effectiveness and the measured effectiveness across students who responded for a student-support in addition to the Sign Test. In comparison to the Sign Test which measures consistent differences, a Chi-Squared Test measures the difference in frequencies between two relationships. A Chi-squared Test was not conducted on the overall effectiveness as the sample size considers all students who were provided the associated student-support which violates the equality of sample sizes across observation. As the results of all the Chi-Squared Tests performed is infeasible to report, only those which found significant results are shown in Table 2.
4. RESULTS
Test Performed | Sample Size | Number of Successful Sign Tests | P-Value | Corrected P-Value |
---|---|---|---|---|
Pair: Overall | 1,699 | 876 | 0.2071 | 0.379 |
Problem: Overall | 1,182 | 607 | 0.3672 | 0.379 |
Pair: Responding Students | 1,320 | 695 | 0.0575 | 0.23 |
Problem: Responding Students | 942 | 485 | 0.379 | 0.379 |
4.1 Overall Effectiveness
As shown in Table 1, across the 5,861 available student-supports with responses, 1,699 pairs of student-supports were created with 876 pairs (proportion estimate of 0.5156) returning a correlated difference on the Sign Test for overall effectiveness. Across the 1,699 pairs of student-supports, there were 1,182 problems, of which 607 (proportion estimate of 0.5135) had a correlated difference on the Sign Test. Both results were not significant () before Benjamini-Hochberg correction.
4.2 Effectiveness Across Responding Students
Also in Table 1, 1,320 pairs of student-supports were created with 695 pairs (proportion estimate of 0.5265) returning a correlated difference on the Sign Test for effectiveness across responding students. Across the 1,320 pairs of student-supports there were 942 problems, of which 485 (proportion estimate of 0.5149) had a correlated difference on the Sign Test. Both results were not significant () before Benjamini-Hochberg correction.
Contingency Table | Expected Table | Critical Statistic | P-Value |
---|---|---|---|
4.8995 | 0.0269 | ||
5.4767 | 0.0193 | ||
4.9383 | 0.0263 | ||
5.5314 | 0.0187 | ||
4.8995 | 0.0269 | ||
8.7625 | 0.0031 |
The Chi-squared Test () revealed only six out of the 5,861 responded student-supports with a relationship between the student perception of effectiveness and the measured effectiveness across the responding students. The results of the significant Chi-squared Tests are shown in Table 2. The significance of the results can be attributed to randomness due to sample size (). The corrected p-values using the Benjamini-Hochberg Procedure[2] also conveys the non-significance of the results at .
5. CONCLUSION
In this work, initial findings show no significant relationship between student perception of effectiveness and the measured effectiveness of a student-support. Only 5% of students who used the original system were considered in the performed analysis. Additionally, less than 1% of the responses collected showed any significance in the relationship. As such, the significance of any current results can be attributed to randomness. The response set is unlikely to be generalizable to other online learning platforms or across students and problems.
Potential improvements to further analysis could view the features of the participating students or problems, such as prior knowledge or problem accuracy. The prompts could also be subdivided further to determine if phrasing has a desired effect on student perception. Accurate markers of student response times could also be recorded to differentiate responses before and after completing the given problem. Additionally, the location of when the prompt was delivered could be moved to better reflect the measure of effectiveness used for an on-demand assistance.
Although no evidence of the relationship between student perception of effectiveness and the measured effectiveness of a student-support was found in this work, other qualities could attempt to better provide understanding of student perceptions on effectiveness in more granular surveys. Future work can explore better opportunities in student perceptions on effectiveness of student-supports for themselves. Afterwards, further steps can be taken to gradually generalize effectiveness overall and eventually to other online learning platforms.
6. ACKNOWLEDGMENTS
We would like to thank the NSF (e.g., 2118725, 2118904, 1950683, 1917808, 1931523, 1940236, 1917713, 1903304, 1822830, 1759229, 1724889, 1636782, & 1535428), IES (e.g., R305N210049, R305D210031, R305A170137, R305A170243, R305A180401, & R305A120125), GAANN (e.g., P200A180088 & P200A150306), EIR (U411B190024 & S411B210024), ONR (N00014-18-1-2768), and Schmidt Futures. None of the opinions expressed here are that of the funders. We are funded under an NHI grant (R44GM146483) with Teachly as a SBIR.
7. REFERENCES
- D. M. Adams, B. M. McLaren, K. Durkin, R. E. Mayer, B. Rittle-Johnson, S. Isotani, and M. Van Velsen. Using erroneous examples to improve mathematics learning with a web-based tutoring system. Computers in Human Behavior, 36:401–411, 2014.
- Y. Benjamini and Y. Hochberg. Controlling the false discovery rate: a practical and powerful approach to multiple testing. Journal of the Royal statistical society: series B (Methodological), 57(1):289–300, 1995.
- J. A. Centra. Student ratings of instruction and their relationship to student learning. American Educational Research Journal, 14(1):17–24, 1977.
- W. G. Cochran. The efficiencies of the binomial series tests of significance of a mean and of a correlation coefficient. Journal of the Royal Statistical Society, 100(1):69–73, 1937.
- W. J. Dixon and A. M. Mood. The statistical sign test. Journal of the American Statistical Association, 41(236):557–566, 1946.
- M. Feng and N. T. Heffernan. Informing teachers live about student learning: Reporting in the assistment system. Technology Instruction Cognition and Learning, 3(1/2):63, 2006.
- T. M. Franke, T. Ho, and C. A. Christie. The chi-square test: Often used and more often misinterpreted. American Journal of Evaluation, 33(3):448–458, 2012.
- N. T. Heffernan and C. L. Heffernan. The assistments ecosystem: Building a platform that brings scientists and teachers together for minimally invasive research on human learning and teaching. International Journal of Artificial Intelligence in Education, 24(4):470–497, 2014.
- N. T. Heffernan and C. L. Heffernan. The assistments ecosystem: Building a platform that brings scientists and teachers together for minimally invasive research on human learning and teaching. International Journal of Artificial Intelligence in Education, 24(4):470–497, 2014.
- J. W. B. Lang and M. Kersting. Regular feedback from student ratings of instruction: Do college teachers improve their ratings in the long run? Instructional Science, 35(3):187–205, May 2007.
- L. Mandouit. Using student feedback to improve teaching. Educational Action Research, 26(5):755–769, 2018.
- B. M. McLaren, T. van Gog, C. Ganoe, M. Karabinos, and D. Yaron. The efficiency of worked examples compared to erroneous examples, tutored problem solving, and problem solving in computer-based learning environments. Computers in Human Behavior, 55:87–99, 2016.
- T. Patikorn and N. T. Heffernan. Effectiveness of crowd-sourcing on-demand assistance from teachers in online learning platforms. In Proceedings of the Seventh ACM Conference on Learning @ Scale, L@S ’20, page 115–124, New York, NY, USA, 2020. Association for Computing Machinery.
- E. Prihar, A. F. Botelho, R. Jakhmola, and I. Heffernan, Neil T. Assistments 2019-2020 school year dataset, Dec 2021.
- E. Prihar and M. Gonsalves. Assistments 2020-2021 school year dataset, Nov 2021.
- E. Prihar, T. Patikorn, A. Botelho, A. Sales, and N. Heffernan. Toward personalizing students’ education with crowdsourced tutoring. In Proceedings of the Eighth ACM Conference on Learning @ Scale, L@S ’21, page 37–45, New York, NY, USA, 2021. Association for Computing Machinery.
- L. M. Razzaq and N. T. Heffernan. To tutor or not to tutor: That is the question. In V. Dimitrova, editor, AIED, pages 457–464. IOS Press, 2009.
- J. Whitehill and M. Seltzer. A crowdsourcing approach to collecting tutorial videos–toward personalized learning-at-scale. In Proceedings of the Fourth (2017) ACM Conference on Learning@ Scale, pages 157–160, 2017.
- J. J. Williams, J. Kim, A. Rafferty, S. Maldonado, K. Z. Gajos, W. S. Lasecki, and N. Heffernan. Axis: Generating explanations at scale with learnersourcing and machine learning. In Proceedings of the Third (2016) ACM Conference on Learning@ Scale, pages 379–388, 2016.
- D. Wood, J. S. Bruner, and G. Ross. The role of tutoring in problem solving. Child Psychology & Psychiatry & Allied Disciplines, 17(2):89–100, 1976.
© 2022 Copyright is held by the author(s). This work is distributed under the Creative Commons Attribution-NonCommercial-NoDerivatives 4.0 International (CC BY-NC-ND 4.0) license.