ABSTRACT
This study explores the relationship between short breaks and student performance as a form of self-regulated learning behavior in K-12 digital learning platforms. Digital learning platforms offer a variety of self-regulated learning tools, such as instructional videos, hints, and scaffolding, which are crucial for self-paced learning. However, these platforms may lack sufficient academic support for students struggling with challenging concepts. The effect of micro-breaks on learning outcomes is not well understood. Building on this goal, we conducted two regression analyses to investigate the number of students who stopped out and returned to finish the assignments and explore the duration of breaks between questions among those students who returned to complete the assignments by examining data from a digital learning platform that covers 6th to 8th-grade mathematics. Specifically, the study investigates the relationship between break intervals and student performance, such as correctness and assignment completion rates. Results reveal that, despite prevalent breaks between problems, break duration does not significantly affect learning performance. The contributions of our research are multifaceted, offering insights that both corroborate and extend previous findings in the realm of student engagement with digital learning platforms.
Keywords
1. INTRODUCTION
Self-regulated learning (SRL) strategies [30, 31] become important concepts in technology-enhanced learning environments such as digital learning platforms [9, 17, 19]. Digital learning platforms have been widely adopted in classroom and other educational settings for the purpose of improving students’ learning and helping teachers to orchestrate their instruction. Many of these platforms supply learners with a range of tools that are designed to guide them in their learning including instructional videos [15], hints and feedback [22], and scaffolding [24]. Similarly, teachers are provided with functions that help them manage and assign content [2], assess student work, and monitor students’ achievements and progress toward learning goals [28], such as hints and instructional videos are available on the learning platform. Demonstrating self-regulated learning behavior on the digital learning platform is one of the factors that contribute to successful learning performance[8, 27, 28], particularly where student-paced progress is supported [8, 26, 28]. Empirical research supports the effectiveness of integrating SRL strategies in digital environments, highlighting their potential to enhance academic performance and foster a more personalized learning experience [13, 15, 23, 27].
However, digital learning platforms sometimes lack providing adequate academic support for students [24]. For example, the limited hints or the videos on the learning platforms may not be enough for students who are struggling with some concepts to build sufficient knowledge to solve the problems. Through scaffolding and feedback provided by human tutors, students find it easier to identify flaws in their reasoning and rectify gaps in their knowledge [25, 24]. When the negative emotions exceed an individual’s limit, students need to step out and seek other help [20, 21], including academic assistance outside the digital learning environment, extra support from teachers, or short breaks.
Students may find it necessary to take a short break when they feel frustrated, temporarily moving away from the learning environment before returning to complete the assignments. We refer to short breaks as micro-breaks in the literature, which refers to a brief interval taken to step away from ongoing work or assignments [16, 5]. In the prior research by Kim, Park, and Niu (2017) [16], the authors discuss micro-break activities that can assist employees in recovering and rejuvenating while they are working. The article by Bosch and Sonnentag (2019) [5] indicates that self-reward can explain the motivation behind taking micro-breaks. The results also indicate that participants tend to take micro-breaks not necessarily when needed but when they desire to reward themselves. However, other work on unproductive persistence, often studied through a behavior known as “wheel spinning” [7], suggests that it may be beneficial for students to step away from assignments if they are struggling and return at a later time. Some learning systems even offer feedback to students to seek help and return the following day if wheel spinning is detected [7, 13]. The duration of the break may be a factor in whether or not it may be beneficial to learning, although little research has been conducted to measure these short break effects within digital learning platforms. It is worth exploring whether the occurrence of short breaks among students on digital learning platforms signifies emotional regulation, seeking additional academic help, or self-reward. Our hypothesis proposes a relationship between the desire for self-reward and the need to take micro-breaks in relation to the learning outcomes.
In addition to "wheel spinning," student attrition which is characterized in some contexts as dropout [14, 29] or stopout [6], is typically considered an unproductive behavior for learning, this may not always be the case. Student attrition in online learning occurs when students fail to receive enough support [3, 12] but may also occur if they achieve their learning goals and see no value in continuing [13, 14, 17, 28], particularly in Massive Online Courses (MOOCs). However, even among those students who exhibit these behaviors in response to insufficient supports, we can further dichotomize this into students who do not return to the content or platform (typically characterized as dropout), and those who do ultimately return (i.e. stopout); in the later case, we may also characterize this behavior as simply the student taking a break from the learning task [3, 17].
As educational technologies and e-learning types grow, research about student attrition and dropout behavior on learning platforms such as MOOCs has been discussed in recent decades [1, 14, 18, 28, 29]. In this study, we refer to the dropout behavior that occurs within a K-12 mathematics learning platform, finding that students are most likely to quit on the first problem of mastery-based assignments [6]. It was further found that there is a notably-disproportionate number of students who stopout before the second problem of these assignments, suggesting that there may be different causes for stopout early in an assignment than are contributing to this behavior later in these assignments. This special case of stopout exhibited on the first problem has been called “refusal” to distinguish this behavior from other forms of attrition.
Refusal behavior, characterized as stopout during or directly following the first problem in an assignment as defined above, has been previously thought to be especially problematic from the perspective of a learning platform as the system is unable to offer help to students who immediately disengage from the content; as many students exhibiting refusal similarly quit after receiving the feedback that they have answered the first problem incorrectly. Prior research on refusal had found the behavior to be more correlated with measures of low self-efficacy than other hypothesized measures such as prior knowledge [6].
One aspect that remains relatively unexplored within the learning platform is the relationship between the length of breaks and other learning outcomes within digital learning platforms. A limitation of that prior work, however, as well as many other works examining stopout, is in the definition of these behaviors that does not consider nor account for students who ultimately return to continue working and often complete the assignments. Understanding whether short breaks between questions enhance students’ learning effectiveness and examining the behavior patterns of students who take breaks and return can help in understanding how well students are able to self-regulate their learning and predicting the attrition and dropout among online learning environments [3, 10, 11, 14, 17, 18, 29].
The goal of this study is to observe whether the duration of breaks among questions in the assignment correlates with higher learning outcomes upon returning and examine whether there are differences in behavioral patterns among students exhibiting different forms of attrition within a learning platform. Building upon prior work which found that students tend to exhibit quitting behavior early within mastery-based assignments [6], we first examine whether similar trends emerge among students who ultimately return to the assignment and those who do not. We then identify students who step away from assignments and return on a different day to examine relationships between the duration of this interval and their performance upon returning. Finally, we examine breaks at a finer granularity by exploring similar relationships for shorter intervals.
Exploring these aspects through the lens of self-regulated learning, characterized by how well students are evidenced to structure their time away from learning content productively, we aim to address the following research questions: 1) Do students take breaks disproportionately on the first problem of an assignment as has been observed among students who exhibit stopout? 2) Does the number of days spend away from an assignment correlate with student performance upon returning to finish their work? 3) For students who struggle on the first problem, do students who take a break tend to exhibit higher correctness upon returning to the assignment? 4) For students who struggle on the first problem, are students who take a break more likely to complete the assignment upon returning?
.png)
2. DATASET
The dataset utilized in this study encompasses data collected from the ASSISTments learning platform which offers various assignment types, including Skill Builders which allow students to answer a series of questions related to a single skill or concept, and mastery is achieved by correctly answering a certain number of questions in a row, typically three. These assignments are assigned by teachers, and completion is determined for each individual student based on their progress towards mastery. The platform implements a failsafe to prevent wheel-spinning behavior [4, 7]; if students are unable to achieve mastery after 10 problems, they are prompted to seek help and return the next day. For this study, our dataset consists of students’ Skill Builder data from 2014 through 2021. The content available on ASSISTments primarily covers 6th to 8th-grade mathematics. The dataset contains interaction logs from 46,766 distinct students across 17,331 unique assignments, totaling 318,752 student–assignment logs. This study was conducted as secondary analysis of de-identified data that had been previously collected for research; the study represents secondary data analysis and is exempt under IRB1.
3. ANALYSIS 1: MULTI-DAY BREAKS
In the initial segment of our analysis, we establish a framework for evaluating students’ learning sessions, specifically addressing breaks that span multiple days. We begin by defining a learning session as the period of student activity within a single day. Any break extending beyond midnight initiates a new session. In this analysis, we further target students who exhibit refusal, where the student starts the first problem on a particular day but does not start the second problem on that same day. This includes students who either exit the system after incorrectly answering their first question or those who quit without attempting any question. For this first analysis, we do filter our data to include only those students who return and complete their assignment in a second session on a later date.
Session duration | |
---|---|
Group 1 | Day 1 |
Group 2 | Day 2-3 |
Group 3 | Day 3-7 |
Group 4 | Day 7 up |
To measure the relationship between the duration of these extended breaks and student performance upon returning, we observe the number of problems completed by the student on their second session as our dependent variable; as these are mastery-based assignments, this number of problems signifies the number of problems attempted by the student to demonstrate sufficient understanding of the material. We categorize students into four distinct groups based on the duration of their time away from the system. The first group comprises students who have a single session on a given day and then return the following day to continue working. The second group consists of students who, after initially stopping out, return to the system after 2-3 days, inclusively. The third and fourth groups represent students who return between 4-7 days later, inclusively, and greater than 7 days following the first session, respectively. This categorization was applied in consideration of the hypothesized non-linear relationship that these sessions may have with our dependent variable.
We apply a linear regression with these categorical variables, using the ‘greater than 7 day’ interval as our reference category, again observing the number of problems completed on the second session as our dependent variable.
Estimate | Std. Error | p | |
---|---|---|---|
Intercept | 4.683 | 0.024 | <.001 *** |
Day 1 | 0.042 | 0.033 | 0.189 |
Day 2-3 | -0.002 | 0.036 | 0.955 |
Day 3-7 | 0.053 | 0.036 | 0.146 |
4. ANALYSIS 2: FINER INTERVALS
Following the approach of Analysis 1, our second analysis focuses on understanding the effects of micro-breaks between questions during a learning session on student performance. This part of our study aims to examine how short intervals, categorized by duration into less than 5 minutes, 5 to 15 minutes, 15 to 30 minutes, and 30 to 60 minutes, correlate with the accuracy of responses and the overall completion of assignments by students.
Using a logistic regression model, we explore the relationship between the duration of micro-breaks and two key measures of student performance: the percent correctness on subsequent questions and assignment completion.
Number of problem logs | Minutes | |
---|---|---|
Group 1 | 1,821,539 | Less than 5 mins |
Group 2 | 1,090 | 5 mins to 15 mins |
Group 3 | 288 | 15 mins to 30 mins |
Group 4 | 207 | 30 mins to 60 mins |
Group 5 | 10,989 | 60 mins up |
Total | 1,834,113 |
In defining the scope of this analysis, we consider only students who answered the first problem incorrectly, and have engaged with at least four questions. As the mastery threshold on these assignments is 3 correct problems in a row, this filtering identifies the students who exhibited struggle on the first problem and subsequently how they performed on the remainder of their assignment; observing beyond the 4th problem would introduce selection biases as certain students would complete their assignment. However it is the case that some students may stopout before this 4th problem, also introducing a risk of bias, which is why we also include completion as a dependent measure for this analysis.
Through this analysis, we seek to uncover patterns suggesting the beneficial effects of well-timed pauses on enhancing student engagement and success. By drawing parallels to the methodology and findings of Analysis 1, this investigation into micro-breaks aims to provide deeper insight into effective learning strategies, particularly highlighting the role of short breaks in helping students overcome initial challenges and achieve academic proficiency. This exploration is crucial for improving our understanding of how temporal dynamics within learning sessions are related to educational outcomes, potentially informing pedagogical strategies to nurture more resilient and adaptable learners.
Estimate | Std. Error | p | |
---|---|---|---|
Intercept | 0.749 | 0.001 | < .001 *** |
5 to 15 minutes | 0.010 | 0.032 | 0.751 |
15 to 30 minutes | 0.031 | 0.068 | 0.653 |
30 to 60 minutes | 0.034 | 0.060 | 0.572 |
> 60 minutes | -0.012 | 0.018 | 0.501 |
Estimate | Std. Error | p | |
---|---|---|---|
Intercept | 2.137 | 0.011 | < .001 *** |
5 to 15 minutes | -0.442 | 0.363 | 0.223 |
15 to 30 minutes | -0.432 | 0.769 | 0.574 |
30 to 60 minutes | -0.122 | 0.753 | 0.871 |
> 60 minutes | -0.210 | 0.223 | 0.347 |
5. RESULTS
In Analysis 1, we employed a linear regression model to examine the relationship between stopout duration and the number of problems needed to achieve mastery upon returning to the assignment over longer time intervals. The results of this linear model are reported in Table 2.
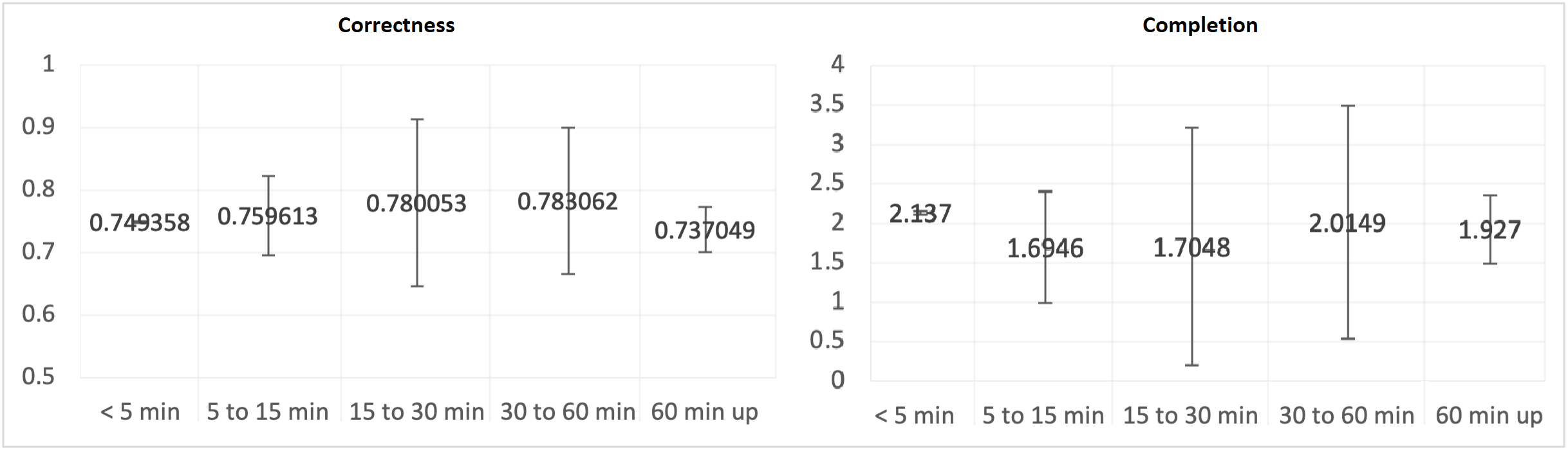
The findings revealed that the duration of the stopout interval did not significantly correlate with the number of problems needed to master the material when they returned to the platform. Compared to the baseline group of students who returned after more than seven days, those who came back to complete their assignments sooner did not show statistically significant differences in their level of completeness. This was evidenced by the p-values, which were all well above the conventional threshold for statistical significance (day 1: p=0.189; day 2-3: p=0.955; day 3-7: p=0.146).
In essence, whether a student returned after one day, a few days, or within a week, the speed (in terms of number of problems) in which they completed their assignments remained statistically indistinguishable from those students who returned after a longer break of more than seven days. This suggests that the time taken for a stopout, within the durations examined, does not have a discernible relationship with students’ completion.
In our exploration of the relationship between micro-breaks and student performance on a learning platform, Analysis 2 utilized a generalized linear model to analyze the patterns of correctness and completion among students who took breaks after encountering difficulties with the first question. This study aimed to discern whether a relationship emerged between the duration of these breaks and subsequent student performance in terms of completion and correctness.
The results, depicted in Table 4 and Figure 2, indicate a negligible trend in the percentage of correctness as the length of the break time increases. The non-overlapping error bars indicate that there is no statistically significant differences observed between the different intervals, although there is a notable trend in terms of variance between them. The data reveals a tendency towards lower correctness for students taking longer breaks, specifically those extending beyond 60 minutes. Conversely, the highest levels of correctness are found following breaks of 15 minutes to an hour, though, again, these results are not statistically significant in comparison to the other intervals.
Table 5 and Figure 2 present the results with regard to completion rates. In this case, intervals shorter than 5 minutes (i.e. arguably non-breaks) seem to be associated with the highest completion rates. Interestingly, a moderate break of 30 to 60 minutes yields a completion rate comparable to that of the shortest breaks, possibly indicating that a well-timed longer break can rejuvenate a student’s focus and commitment to task completion. However, similar to correctness, the variance indicated by the error bars suggests these findings are not statistically significant, and thus should be interpreted with caution.
6. DISCUSSION AND LIMITATION
In the synthesis of our findings from Analyses 1 and 2, our analyses attempted to discern whether the durations of stopouts and micro-breaks correlate with several learning outcomes in the context of mastery learning assignments.
Analysis 1 employed a linear regression model, which did not corroborate our initial hypothesis that longer stopouts would correlate with a decreased number of problems required to achieve mastery upon a student’s return. The p-values associated with varying durations of stopout—- be it a day, a few days, or up to a week—- did not meet the conventional thresholds for statistical significance. These outcomes indicate that the time span of a student’s disengagement from the platform does not materially affect their speed in completing assignments upon their return.
Analysis 2 extended our examination to micro-breaks, the brief intervals taken between individual questions. The patterns observed in correctness and completion metrics revealed no statistically significant differences across varying break lengths, although certain trends emerged. Students taking longer breaks, particularly beyond 60 minutes, showed a tendency toward lower correctness, whereas moderate breaks of 15 to 30 minutes and 30 to 60 minutes were associated with higher correctness and completion rates. Despite these trends, the non-significant p-values necessitate a cautious interpretation of these findings.
The results across both analyses did not align with our initial hypotheses, suggesting that factors beyond the scope of our current measures may be influencing student performance. This may include qualitative aspects of how students spend their time away from the platform or systemic factors such as academic schedules and periods that dictate when breaks occur. The observed stopout behavior is consistent with self-regulated learning strategies, where students consciously decide when to take breaks. This pattern of disengagement, particularly after an incorrect response, may represent an adaptive strategy, allowing time for reflection or stress alleviation before re-engaging with the material. The potential benefits of such strategic pauses, while not quantitatively validated in our study, resonate with the principles of self-regulated learning, suggesting that even non-significant trends may hold pedagogical value.
We acknowledge there are some limitations of this study. This study was confined to examining specific mathematical assignments within a particular academic year, which may limit the generalizability of our findings. In addition, our analyses implemented several filtering steps to identify a very specific subset of student behaviors that had not previously been identified or well-studied in prior work. This selection criterion was intended to capture data from students demonstrating a certain level of engagement and to observe behaviors indicative of mastery on the platform. Another limitation arises from the variable difficulty levels of the assignments. The complexity of tasks may significantly influence student performance and engagement, and our study did not control for these variations nor examine causal relationships. The recognition of differing difficulty levels across assignments suggests that they could play a confounding role in our analysis of stopout and breaks.
Future research endeavors could also aim to collect and analyze data that captures the qualitative aspects of students’ time spent during breaks. Such information could shed light on the activities students engage in during their disengagement from the platform and how these may affect their subsequent performance. Additionally, exploring the systemic reasons behind why students take breaks, such as the end of a school period or external distractions, could provide further insights into how these factors interact with self-regulated learning behaviors.
While our study did not uncover statistically significant relationships between break durations and performance metrics, it did provide evidence of student behaviors that align with self-regulated learning strategies. These insights contribute to an emerging narrative that emphasizes the importance of strategic pauses in enhancing the efficacy of learning in digital contexts. Further investigation into the underlying factors influencing these behaviors and their potential impacts on learning could inform the design of digital learning platforms to better support student success.
7. CONCLUSION
By conducting a comparison with prior research on student refusal behavior [6], our work has provided further evidence of the presence of disproportionate stopout rates on the first problem of mastery-based assignments. Our study contributes to the existing literature by delving into the nuanced dimensions of stopout behavior. We advance the understanding of how both prolonged absences and micro-breaks may relate to students’ performance. While the findings from our regression analyses did not yield statistically significant results, they revealed trends that suggest different intervals may exhibit different variances in terms of learning outcomes at the very least. By examining the frequency of stopout behavior among students who eventually return to their tasks, we have also expanded the narrative around self-regulated learning strategies. Our findings suggest that a subset of students may employ stopout as a strategic maneuver, potentially to seek remedial help or engage in reflective pauses, thus showcasing proactive engagement with the learning material. This aligns with the self-regulation theory and underscores the role of strategic pauses in effective learning.
In conclusion, by understanding the patterns and implications of stopout and refusal behaviors, educators and platform developers can design more effective and adaptive learning environments that support students’ needs for cognitive rest, reflection, and engagement with learning tasks.
8. ACKNOWLEDGMENTS
We would like to thank NSF (e.g., 2331379, 1903304, 1822830, 1724889), as well as IES (R305B230007), Schmidt Futures, MathNet, and OpenAI.
9. REFERENCES
- M. Adnan, A. Habib, J. Ashraf, S. Mussadiq, A. A. Raza, M. Abid, M. Bashir, and S. U. Khan. Predicting at-risk students at different percentages of course length for early intervention using machine learning models. Ieee Access, 9:7519–7539, 2021.
- M. Andergassen, G. Ernst, V. Guerra, F. Mödritscher, M. Moser, G. Neumann, and T. Renner. The evolution of e-learning platforms from content to activity based learning: The case of learn@ wu. In 2015 International Conference on Interactive Collaborative Learning (ICL), pages 779–784. IEEE, 2015.
- L. M. Angelino, F. K. Williams, and D. Natvig. Strategies to engage online students and reduce attrition rates. Journal of Educators Online, 4(2):n2, 2007.
- M. Boekaerts. Self-regulated learning: A new concept embraced by researchers, policy makers, educators, teachers, and students. Learning and instruction, 7(2):161–186, 1997.
- C. Bosch and S. Sonnentag. Should i take a break? a daily reconstruction study on predicting micro-breaks at work. International Journal of Stress Management, 26(4):378, 2019.
- A. F. Botelho, A. Varatharaj, E. G. V. Inwegen, and N. T. Heffernan. Refusing to try: Characterizing early stopout on student assignments. In Proceedings of the 9th international conference on learning analytics & knowledge, pages 391–400, 2019.
- A. F. Botelho, A. Varatharaj, T. Patikorn, D. Doherty, S. A. Adjei, and J. E. Beck. Developing early detectors of student attrition and wheel spinning using deep learning. IEEE Transactions on Learning Technologies, 12(2):158–170, 2019.
- J. Broadbent and W. L. Poon. Self-regulated learning strategies & academic achievement in online higher education learning environments: A systematic review. The internet and higher education, 27:1–13, 2015.
- A. Chelghoum. Promoting students’ self-regulated learning through digital platforms: New horizon in educational psychology. American Journal of Applied Psychology, 6(5):123–131, 2017.
- K. Coussement, M. Phan, A. De Caigny, D. F. Benoit, and A. Raes. Predicting student dropout in subscription-based online learning environments: The beneficial impact of the logit leaf model. Decision Support Systems, 135:113325, 2020.
- F. Del Bonifro, M. Gabbrielli, G. Lisanti, and S. P. Zingaro. Student dropout prediction. In Artificial Intelligence in Education: 21st International Conference, AIED 2020, Ifrane, Morocco, July 6–10, 2020, Proceedings, Part I 21, pages 129–140. Springer, 2020.
- S. D’Mello and A. Graesser. Dynamics of affective states during complex learning. Learning and Instruction, 22(2):145–157, 2012.
- R. S. Jansen, A. van Leeuwen, J. Janssen, R. Conijn, and L. Kester. Supporting learners’ self-regulated learning in massive open online courses. Computers & Education, 146:103771, 2020.
- C. Jin. Mooc student dropout prediction model based on learning behavior features and parameter optimization. Interactive Learning Environments, 31(2):714–732, 2023.
- G. Johnson and S. Davies. Self-regulated learning in digital environments: Theory, research, praxis. British Journal of Research, 1(2):1–14, 2014.
- S. Kim, Y. Park, and Q. Niu. Micro-break activities at work to recover from daily work demands. Journal of Organizational Behavior, 38(1):28–44, 2017.
- R. F. Kizilcec and S. Halawa. Attrition and achievement gaps in online learning. In Proceedings of the second (2015) ACM conference on learning@ scale, pages 57–66, 2015.
- A. Kukkar, R. Mohana, A. Sharma, and A. Nayyar. Prediction of student academic performance based on their emotional wellbeing and interaction on various e-learning platforms. Education and Information Technologies, pages 1–30, 2023.
- T. Lehmann, I. Hähnlein, and D. Ifenthaler. Cognitive, metacognitive and motivational perspectives on preflection in self-regulated online learning. Computers in human behavior, 32:313–323, 2014.
- R. Pekrun. The control-value theory of achievement emotions: Assumptions, corollaries, and implications for educational research and practice. Educational psychology review, 18:315–341, 2006.
- R. Pekrun, S. Lichtenfeld, H. W. Marsh, K. Murayama, and T. Goetz. Achievement emotions and academic performance: Longitudinal models of reciprocal effects. Child development, 88(5):1653–1670, 2017.
- J. Schwerter, F. Wortha, and P. Gerjets. E-learning with multiple-try-feedback: Can hints foster students’ achievement during the semester? Educational technology research and development, 70(3):713–736, 2022.
- K. Steffens. Self-regulated learning in technology-enhanced learning environments: Lessons of a european peer review. European journal of education, 41(3-4):353–379, 2006.
- K. VanLehn. The relative effectiveness of human tutoring, intelligent tutoring systems, and other tutoring systems. Educational psychologist, 46(4):197–221, 2011.
- K. VanLehn, S. Siler, C. Murray, T. Yamauchi, and W. B. Baggett. Why do only some events cause learning during human tutoring? Cognition and Instruction, 21(3):209–249, 2003.
- K. Wijekumar, L. Ferguson, and D. Wagoner. Problems with assessment validity and reliability in web-based distance learning environments and solutions. Journal of Educational Multimedia and Hypermedia, 15(2):199–215, 2006.
- F. I. Winters, J. A. Greene, and C. M. Costich. Self-regulation of learning within computer-based learning environments: A critical analysis. Educational psychology review, 20:429–444, 2008.
- J. Wong, M. Baars, D. Davis, T. Van Der Zee, G.-J. Houben, and F. Paas. Supporting self-regulated learning in online learning environments and moocs: A systematic review. International Journal of Human–Computer Interaction, 35(4-5):356–373, 2019.
- Y. Zhou, J. Zhao, and J. Zhang. Prediction of learners’ dropout in e-learning based on the unusual behaviors. Interactive Learning Environments, 31(3):1796–1820, 2023.
- B. J. Zimmerman. A social cognitive view of self-regulated academic learning. Journal of educational psychology, 81(3):329, 1989.
- B. J. Zimmerman. Self-regulated learning and academic achievement: An overview. Educational psychologist, 25(1):3–17, 1990.
1This study was conducted with de-identified data shared and analyzed under University of Florida IRB202102682