ABSTRACT
A widely used minimum criterion for intelligent tutoring system (ITS) status is that the system provides within-problem support. The ITS literature abounds with comparisons of supports, but investigations on the transferability of findings, particularly between education levels, are scant. There are, however, significant impetuses to investigate the transferability of these findings. First, ITSs are used throughout K-16, and findings from past studies naturally serve as guideposts for subsequent ITS developments and research. If findings do not transfer, these guideposts may be illusory. Furthermore, learners change over time, and the efficacy of ITS supports that do not adapt to these changes may vary. This study conceptually replicates investigations conducted at the middle school level by assigning 330 college students in introductory mathematics courses homework that have the same within-problem support formats. The support formats are either text hints, text explanations, or video explanations. We compare the efficacy of these support formats within the college student sample and between college student and middle school student samples. Our findings at the college level indicate that on-demand within-problem explanations displayed as text rather than videos lead to higher next problem correctness and that both outperform text hints. Our results differ from those in the literature using middle school student samples and therefore buttress the assertion that studies investigating the transferability of findings between education levels are necessary.
Keywords
INTRODUCTION
A widely used criterion for intelligent tutoring system (ITS) status is that the system has an inner loop [1], i.e., provides within-problem supports and not just end-of-problem feedback. The ITS literature abounds with comparisons of supports, including scaffolding versus hints [2], worked examples versus erroneous examples [3], and the supports which will be compared in this study, namely video hints versus text hints [4] and explanations versus hints [5]. Many ITS studies that evaluate the efficacy of these supports commonly involve a single segment of the student population, e.g., middle school students. Investigations on the transferability of findings, particularly to college student, are scant.
There are, however, significant impetuses to investigate the transferability of findings to other segments of the student population. First, ITSs are used throughout K-16, and findings from past studies naturally serve as guideposts for subsequent ITS developments and research. If findings do not transfer, these guideposts may be illusory. There is evidence to believe this is the case since [6] considered if the findings in [2] transferred to adult learners in a MOOC and found that the findings between hints and scaffolds did not transfer. Therefore, learners change over time, and the efficacy of ITS supports that do not adapt to these changes may vary.
For example, when describing what can be considered a good hint in an ITS, [7] in part states “Hints should abstractly (but succinctly) characterize the problem-solving knowledge (Anderson et al., 1995), by stating in general terms both the action to be taken, the conditions under which this particular action is appropriate, and the domain principle (e.g., geometry theorem) that justifies the action.” However, [8] asserted that young adolescents between the ages of 10-15 are in a transition period from concrete thinking to abstract thinking. Findings in [9] support this assertion and showed that middle school students benefited more from concepts with which they could directly relate, whereas college students benefited more from abstract concepts. This is relevant to within-problem supports while solving a mathematics problem. Hints which abstractly characterize the problem-solving knowledge, state in general terms the action to be taken and the conditions under which it is appropriate as [7] suggests may better serve college students who have the cognitive ability to assimilate and apply theorems and abstract definitions, whereas middle school students may be better served by an exposition through relatable or worked examples.
Similarly, a significant consideration in any instructional setting is Cognitive Load Theory (CLT) which stipulates that some difficulties in learning are due to unnecessary and extraneous working memory load. Here again, research has grappled with worked examples [10], including in comparison to tutored problem solving [11] and in ITSs [12, 13]. For example, the redundancy principle builds on the idea that learners utilize distinct information processing channels to internalize information, and hence stipulates that information should not be conveyed through multiple channels simultaneously to avoid depressing the intake from both channels and hampering learning. Research rooted in CLT at the middle school level compared the delivery medium of feedback messages within an ITS and stated that information is better internalized and learning gains are greater when feedback is presented as a video instead of text [4]. However, recent research suggests that executive function skills, which include monitoring and manipulating information in mind (working memory), suppressing distracting information and unwanted responses (inhibition), and flexible thinking (shifting), play a critical role in the development of mathematics proficiency [14]. Although the literature is limited in terms of disentangling the neurodevelopmental aspects of specific cognitive processes in relation to their impact on arithmetic skill development, the research by [15] shows that with development there is a shift from prefrontal cortex-mediated information processing to more specialized mechanisms in the posterior parietal cortex. Freeing the prefrontal cortex from computational load and thus making available valuable processing resources for more complex problem solving and reasoning is a key factor in mathematical learning and skill acquisition [16]. According to [17], some of these processes, especially decision making and working memory, have protracted developmental timelines, but large changes can be detected in relatively small timeframes including from the beginning of middle school to the end of middle school [18, 19]. Therefore, when devising pedagogical supports, it is important to consider the differential effects on cognitive load depending on the student’s attainment of neurodevelopmental milestones and subject matter fluency.
In this study, we break ground on investigating the transferability of findings from the middle school level to the college level regarding the efficacy of within-problem supports for mathematics ITSs. Specifically, this study conceptually replicates investigations conducted at the middle school level by assigning college students homework problems that have the same within-problem support formats. The support formats are either text hints, text explanations, or video explanations. We compare the efficacy of these support formats within the college student sample, and between college student and middle school student samples. For the within college sample investigation, we hypothesize that the findings from the replicated studies will transfer. For the between educational level investigation, we hypothesize that on average college student performance on the subsequent similar question will be similar given the support format (i.e., the relative frequency that the initial action is the correct solution will be equal).
Thus, we pose the following research questions:
- Does the format of within-problem support impact learning outcomes for college students?
- Are learning outcomes the same for college students and middle school students, given the within-problem support format?
Background
For this study, we used ASSISTments which is a free web-based platform that allows teachers to choose from thousands of problems, each of which can provide immediate feedback and support. This support can be customized, e.g., it can be hint messages conveyed as either text or videos, explanations of step-by-step solutions, correctness feedback, etc. One facet of the ASSISTments ecosystem is E-TRIALS, which allows teachers and external researchers to create randomized control trials (RCTs) that run in ASSISTments to test the efficacy of supports.
The literature contains numerous RCTs using E-TRIALS which compare the efficacy of supports. To investigate the transferability of findings from the middle school level to the college level regarding the efficacy of supports, we will next briefly discuss the studies which we conceptually replicate.
Explanations Versus Hints
Cognitive scientists have investigated the role of worked examples and explanations in reducing cognitive load, and there have been several studies on their effectiveness [10, 12]. On the other hand, several studies in the literature have found evidence of the benefit of greater interaction in tutored problem-solving in ITSs [12, 13], including for less proficient students [11].
[5] investigated whether students in a classroom setting benefit more from interactive tutored problem solving than from worked examples given as a feedback mechanism. For the experiment, researchers created nine problem sets, each consisting of four to five ASSISTments. The ASSISTments questions were taken from 6th Grade MCAS tests for Mathematics (2001 – 2007) focusing on the Patterns, Relations and Algebra section, which concentrates on populating a table from a relation, finding a missing value in a table, using fact families, determining equations for relations, substituting values into variables, interpreting relations from number patterns, and finding values from a graph.
In their study, 186 8th grade students from three middle schools participated. Over 80% of students who participated were from a school that had state test scores in the bottom 5% of that state. Results from this study show that there was a significant effect for the condition with tutored problem solving receiving higher gain scores than worked examples (35% versus 13% average gain), t(67) = 2.38, p = 0.02.
Video Versus Text
Prior research has found that dynamic graphics are more effective than static graphics in mathematics realms [20], and videos and web-based homework support have grown increasingly popular in educational settings [21]. Some research suggests that video is not universally successful in promoting learning gains [22]. [4] assess the effects of feedback medium within a problem set derived from preexisting ASSISTments certified material. The structure of the problem set relied on three questions with text feedback and three isomorphic questions with video feedback and was presented to students in fixed question patterns to allow all students an equivalent opportunity to experience both feedback styles. Video content mirrored textual feedback to provide identical assistance through both mediums.
139 8th grade students from four classes comprised of four classes that spanned four suburban middle schools participated in this study. After exclusion criteria, 89 remained for analysis. The study’s primary analysis assessed student performance on the second question as a function of the feedback medium they experienced after incorrectly answering the first question. As will be again addressed in the discussion section, learning outcomes were not enhanced for students who received video feedback rather than text feedback. There was also no significant difference in the overall number of feedback levels experienced by students.
Methodology
Procedure
330 college students in 6 sections of introductory mathematics courses at two institutions participated in this study. Demographic information of this group is as follows: 9% transfer students, 56% female, 44% male, 52% White, 19% Black, 16% Hispanic, 7 % Asian, 4% two or more races, 1% International, and 1% Unknown.
Students were assigned up to 5 problems sets (PS) as homework during the last three weeks of the Fall 2021 semester in a manner that was consistent with regular educational practices. Each problem set covered one topic, namely rational exponents (PS 1), multiplying and dividing monomials (PS 2), scientific notation (PS 3), writing linear equations given ordered pairs (PS 4), and systems of linear equations (PS 5). A problem set was only assigned to a class if it fell within the course’s curriculum.
Students were free to work at their own pace and were not required to complete the assignments in one sitting. Log data was recorded for each student’s actions, including first action, correctness, response time, attempts, hint requests, and feedback type, and this data was received from The ASSISTments Foundation.
Design
The five problem sets were made using preexisting ASSISTments certified material. Problem sets 1-3 consisted of 30 problems each, and problem sets 4 and 5 consisted of 20 problems each. Problems had between 1 and 9 parts, for a combined total of 196 parts in all problem sets. Each part had exactly one input field which required a free-response answer usually consisting of a number or mathematical expression. Questions within a problem set were assigned in random order to eliminate potential ordering effects.
Students could attempt any part an unlimited number of times until they entered the correct answer. Each part had on-demand within-problem supports consisting entirely of either text hints, text explanations, or video explanations. Students were aware that there were different kinds of support types but did not know which types of support any particular question had. If a student requested within-problem support, we relied on ASSISTments to randomly assign the student one of the available supports.
Our analysis assesses student performance on the question part immediately after the question part for which support was requested, which we will refer to as the next question and previous question, respectively. As such, one of our exclusion criteria is that any problem for which a student did not complete the next question is omitted. We assess student performance based on the next problem’s correctness which we refer to as learning outcomes. To that end, we consider the next question to be incorrect if a student’s first action is to either submit a wrong solution or request support.
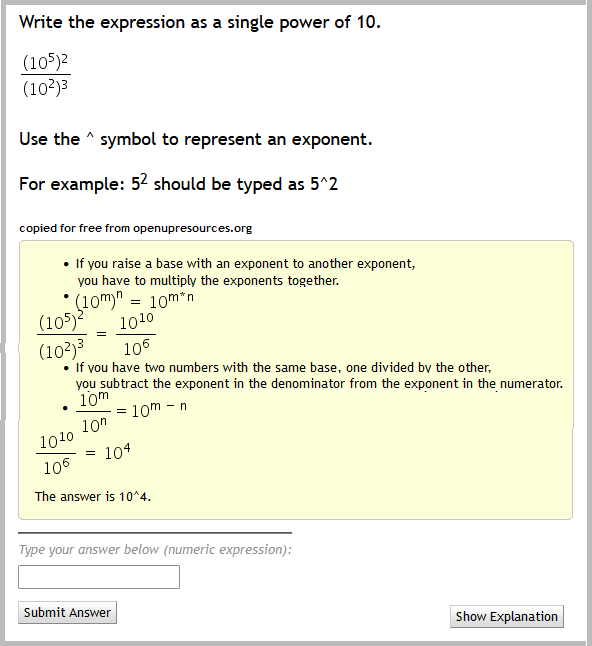
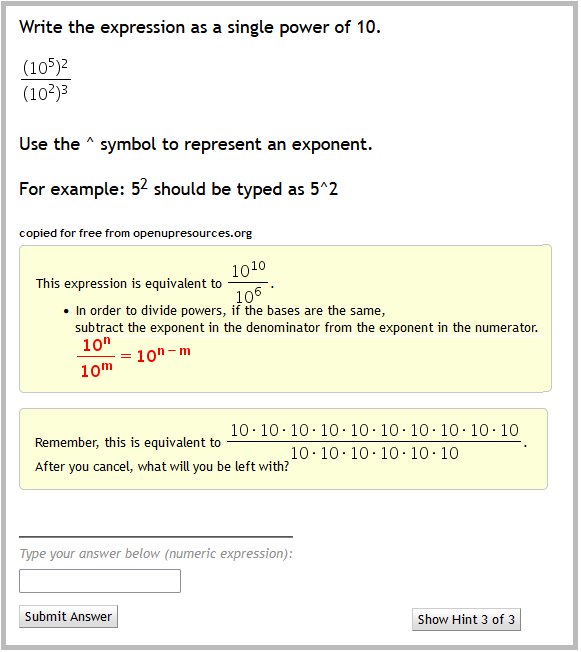
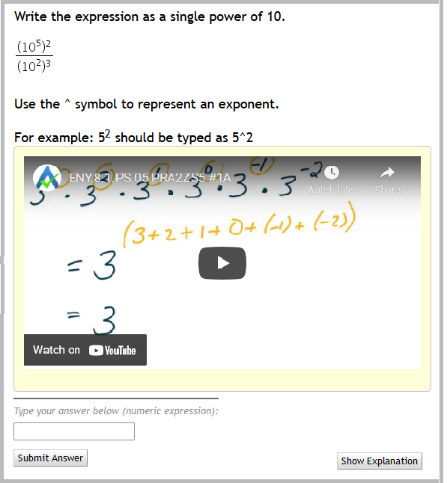
Results
Next Question Performance Analysis Within College Student Sample
To address our first research question, we assessed the next question performance of students who received feedback on the previous question. In total, 40126 solution steps were completed by students included in our analysis, and on 4820 solution steps (4820/40126 = 0.120) supports were provided, as Table 1 shows.
Learning outcomes were more enhanced for students who received text explanations ( = 0.493, SD = 0.009) rather than video explanations ( = 0.390, SD = 0.018), and the difference in proportions of 0.103 was statistically significant (p << 0.01). This indicates that students answered the next question correctly over 26 percent more often (0.493/0.390 = 1.264) after receiving on-demand within-problem text explanations than video explanations.
Support Format 1 | Support Format 2 | Difference in Proportions | 95% Confidence Interval for Difference in Proportions |
---|---|---|---|
Text Explanation (N = 3161) | Video Explanation (N = 757) | ||
0.493 (0.009) | 0.390 (0.018) | 0.103* | [0.064, 0.142] |
Text Explanation (N = 3161) | Text Hints (N = 902) | ||
0.493 (0.009) | 0.135 (0.011) | 0.358* | [0.329, 0.386] |
Video Explanation (N = 757) | Text Hints (N = 902) | ||
0.390 (0.018) | 0.135 (0.011) | 0.254* | [0.213, 0.296] |
Note: Performance metrics are depicted as mean (standard deviation). *p << 0.01.
Learning outcomes were enhanced for students who received text explanations rather than text hints ( = 0.135, SD = 0.011). The difference in proportions of 0.358 was statistically significant (p << 0.01) indicating that students were over 3.5 times more likely (0.493/0.135 = 3.652) to answer the next question correctly after receiving ext explanations than text hints.
Learning outcomes were enhanced for students who received video explanations rather than text hints, and the difference in proportions of 0.254 was statistically significant (p << 0.01). This indicates that students were nearly 3 times more likely (0.390/0.135 = 2.889) to answer the next question correctly after receiving on-demand within-problem video explanations than text hints.
discussion
Comparison of Next Question Performance Between Education Levels
To address our second research question, we compare the enhancements of learning outcomes for middle school students to those from our college student sample using the combinations of support formats in [4] and [5].
Before drawing comparisons to our results, it should first be noted that the statistical analysis in [4] has apparent flaws based on the data provided in that paper, and these flaws affect the conclusion of that study. After correcting those analysis errors using the data the authors provided in [4], the correct conclusion of [4] should be that the modality of the support in that study did not have a statistically significant effect on learning outcomes. Nevertheless, the results of [4] are only partially reflected in our results. That is, college students were nearly 3 times more likely to answer the next question correctly after receiving on-demand within-problem video explanations rather than text hints, but over 26 percent more likely to answer the next question correctly after receiving on-demand within problem text explanations rather than video explanations. As previously indicated, both results are also statistically significant.
Another important difference to note is the magnitudes of the efficacies of the different forms of support. At the middle school level, [4] found that students who received video support answered the next question correctly 76% of the time on average, whereas in our study students who received video support answered the next question correctly only 39% of the time on average, with a 95% confidence interval of [0.354, 0.426]. Furthermore, [4] found that middle school students who received text hints answered the next question correctly 52% of the time on average, whereas in our study students who received text hints answered the next question correctly only 13.5% of the time on average, with a 95% confidence interval of [0.113, 0.157]. Therefore, the results in [4] fall well outside of our expected range.
[5] found that learning outcomes were greater for middle school students who received tutored problem solving rather than worked examples and that this difference was statistically significant. This is not reflected in our results since college students were over 3.5 times more likely to answer the next question correctly after receiving on-demand within-problem text explanations than text hints. As previously indicated, the results from our sample are also statistically significant.
Since the results in [5] are presented as average learning gains from a pre- to a post-test, and our results are given as next problem correctness, we cannot draw similar point estimate comparisons to that study.
Contributions, Limitations, and Future Research
We make a significant contribution by breaking ground on investigating the transferability of findings from the middle school level to the college level regarding the efficacy of on-demand within-problem supports for mathematics ITSs. The ITS literature abounds with investigations using on-demand within-problem supports, particularly for middle school and high school students. Some studies have compared the efficacy of these supports and have led to what has been coined “Best So Far” supports – those supports which have led to the highest measures of learning outcomes for comparable studies [23, 24]. Our conceptual replication of these studies with college students did not support the same hierarchy of supports nor magnitude of efficacy at the college level.
This beckons the question, “Best So Far” for whom? To that end, our study identifies a rich area of research since there are abundant ITS studies that often include only one segment of the student population. While it is understandably hard to include students from several education levels in a study, particularly for mathematics ITSs, our results buttress the assertion that studies investigating the transferability of findings between education levels are necessary. Our study also lends credence to the importance of considering who is or is not included in a study, and disaggregating results when possible to ensure inclusion, diversity, equity, and accessibility in research and practice.
Another rich area of research is to data-mine the results from such transferability studies to supplement the limited literature on neurodevelopmental aspects of specific cognitive processes in relation to their impact on learning and best practices for instruction.
Future iterations in this line of research should consider a closer replication of the literature. While our study investigated the modality effects of on-demand within-problem supports, we compared video explanations to text explanations, while [4] compared video hints to text hints. Similarly, while our study compared the impact of interactive forms of tutored problem solving to static forms of support, our study used text explanations and text hints, whereas [5] used worked examples and text hints.
Future research should also consider that while in this study problem sets were only assigned to a class if they fell within the course’s curriculum, a potential concern is that likely exposure to these topics in prior courses may lead to a college student being more familiar with the topics in the problem sets than middle school students, resulting in a confound that could influence our results. Possible indicators of this would be fewer support requests from college students, or higher measures of next problem correctness. Our results indicate the opposite. As was previously shown, college students requested support on 12 percent of solution steps (4820/40126 = 0.120), but in [4] middle school students requested support on approximately 8 percent of solution steps (60/720 = 0.083). Furthermore, as was also previously shown, next problem correctness was consistently lower for college students than for middle school students when comparing similar supports.
On the other hand, since the assignment topics in the original studies differ from each other and from those used in this study, it can be argued that the difficulty of these topics may also differ, again leading to a confound. This too may be indicated by different levels of support requests or different levels of next problem correctness. Based on the just presented discussion, one conclusion which could be drawn from our results may indicate that the topics covered in the problem set by the college students were more difficult than those completed by middle school students. Another conclusion could be that the problems in assignments completed by college students were equally difficult to those completed by middle school students and that the overall relative mathematics skill levels of the two groups differed.
Disentangling these results further is not possible using the results of our study or the available information from the replicated studies. While challenging to implement, something that could mitigate this ambiguity is for future research to include a measure comparing mathematics abilities across education levels, e.g., a pretest, and assign identical problem sets to all study participants across education levels. Furthermore, by including a posttest, future research should also further analyze other learning outcomes, such as that of [5].
Finally, an exclusion criterium in this study is that any problem for which the student did not complete the next question is omitted, and therefore attrition may not have been entirely random. While we do not believe this had any substantial impact on our results, future research should definitively ensure this is true by establishing a more controlled experimental environment.
Acknowledgements
We would like to thank The ASSISTments Foundation. As always, K.H.S., J.M.S., E.M.S., and W.J.S. thank you and I l. y.
REFERENCES
- VanLehn, K., 2006. The behavior of tutoring systems. International journal of artificial intelligence in education, 16(3), pp.227-265.
- Razzaq, L. and Heffernan, N.T., 2006, June. Scaffolding vs. hints in the Assistment System. In International Conference on Intelligent Tutoring Systems (pp. 635-644). Springer, Berlin, Heidelberg.
- McLaren, B.M., van Gog, T., Ganoe, C., Karabinos, M. and Yaron, D., 2016. The efficiency of worked examples compared to erroneous examples, tutored problem solving, and problem solving in computer-based learning environments. Computers in Human Behavior, 55, pp.87-99.
- Ostrow, K. and Heffernan, N., 2014, July. Testing the multimedia principle in the real world: a comparison of video vs. Text feedback in authentic middle school math assignments. In Educational Data Mining 2014.
- Shrestha, P., Maharjan, A., Wei, X., Razzaq, L., Heffernan, N.T. and Heffernan, C., 2009. Are worked examples an effective feedback mechanism during problem solving. In Proceedings of the 31st annual conference of the cognitive science society (pp. 1294-1299).
- Zhou, Y., Andres-Bray, J.M., Hutt, S., Ostrow, K., Baker, R. S., 2021. A Comparison of Hints vs. Scaffolding in a MOOC with Adult Learners. In Roll, I., McNamara, D., Sosnovsky, S., Luckin, R., Dimitrova, V. (eds) Artificial Intelligence in Education. AIED 2021. Lecture Notes in Computer Science (), vol 12749. Springer, Cham. https://doi.org/10.1007/978-3-030-78270-2_76
- Aleven, V., Roll, I., McLaren, B.M. and Koedinger, K.R., 2016. Help helps, but only so much: Research on help seeking with intelligent tutoring systems. International Journal of Artificial Intelligence in Education, 26(1), pp.205-223.
- National Middle School Association and Believe, T.W., 1995. Developmentally Responsive Middle Level Schools. A Position Paper of the National Middle School Association. NMSA. Columbus, Ohio: NMSA.
- Song, H.D., Grabowski, B.L., Koszalka, T.A. and Harkness, W.L., 2006. Patterns of instructional-design factors prompting reflective thinking in middle-school and college level problem-based learning environments. Instructional Science, 34(1), pp.63-87.
- Chi, M.T., Siler, S.A., Jeong, H., Yamauchi, T. and Hausmann, R.G., 2001. Learning from human tutoring. Cognitive science, 25(4), pp.471-533.
- Razzaq, L., Heffernan, N.T. and Lindeman, R.W., 2007. What level of tutor feedback is best. In Proceedings of the 13th Conference on Artificial Intelligence in Education, IOS Press.
- Graesser, A.C., Moreno, K., Marineau, J., Adcock, A., Olney, A., Person, N. and Tutoring Research Group, 2003. AutoTutor improves deep learning of computer literacy: Is it the dialog or the talking head. In Proceedings of artificial intelligence in education (Vol. 4754).
- Graesser, A., Jackson, G.T., Jordan, P., Olney, A., Rose, C.P. and vanLehn, K., 2005. When is reading just as effective as one-on-one interactive human tutoring?. In Proceedings of the Annual Meeting of the Cognitive Science Society (Vol. 27, No. 27).
- Cragg, L. and Gilmore, C., 2014. Skills underlying mathematics: The role of executive function in the development of mathematics proficiency. Trends in neuroscience and education, 3(2), pp.63-68.
- Menon, V., 2010. Developmental cognitive neuroscience of arithmetic: implications for learning and education. Zdm, 42(6), pp.515-525.
- Van Merrienboer, J.J. and Sweller, J., 2005. Cognitive load theory and complex learning: Recent developments and future directions. Educational psychology review, 17(2), pp.147-177.
- Kwon, H., Reiss, A.L. and Menon, V., 2002. Neural basis of protracted developmental changes in visuo-spatial working memory. Proceedings of the National Academy of Sciences, 99(20), pp.13336-13341.
- Viterbori, P., Usai, M.C., Traverso, L. and De Franchis, V., 2015. How preschool executive functioning predicts several aspects of math achievement in Grades 1 and 3: A longitudinal study. Journal of Experimental Child Psychology, 140, pp.38-55.
- Holmes, J. and Adams, J.W., 2006. Working memory and children’s mathematical skills: Implications for mathematical development and mathematics curricula. Educational Psychology, 26(3), pp.339-366.
- Mayer, R.E. (Ed). 2005. The Cambridge handbook of multimedia learning. New York: Cambridge University Press
- Kelly, K., Heffernan, N., D'Mello, S., Namias, J., & Strain, A. 2013. Adding teacher-created motivational video to an ITS. In Proceedings of the Florida Artificial Intelligence Research Society Conference (FLAIRS 2013). 503-508.
- Pane, J.F. 1994. Assessment of the ACSE science learning environment and the impact of movies and simulations. Carnegie Mellon University, School of Computer Science Technical Report CMU-CS-94-162, Pittsburgh, PA.
- Patikorn, T. and Heffernan, N.T., 2020, August. Effectiveness of crowd-sourcing on-demand assistance from teachers in online learning platforms. In Proceedings of the Seventh ACM Conference on Learning@ Scale (pp. 115-124).
- Prihar, E., Patikorn, T., Botelho, A., Sales, A. and Heffernan, N., 2021, June. Toward Personalizing Students' Education with Crowdsourced Tutoring. In Proceedings of the Eighth ACM Conference on Learning@ Scale (pp. 37-45).
© 2022 Copyright is held by the author(s). This work is distributed under the Creative Commons Attribution-NonCommercial-NoDerivatives 4.0 International (CC BY-NC-ND 4.0) license.