ABSTRACT
Twitter communities receive increased attention as informal environments for teacher professional development. However, the diversity and temporal evolution in user adoption, switching, and retention are understudied. This study uses the diffusion of innovation (DOI) framework to examine user switching patterns in two large educational Twitter communities (N = 2,039,260 tweets, N = 104,847 unique users). We find that users’ DOI types relate to user switching over time. Features of the DOI user classifications and other user-level characteristics explain 79% of the variance in user switching decisions and 15% of the timing of user switching. In particular, community switching depends on users’ community entry time, Twitter account age, community interaction centrality, and user type (i.e., teacher vs. non-teacher). Therefore, users’ perceived community fit and their relative motivation to seek out novel communities to engage with can help explain community-switching behavior. Overall, this study informs community-level interventions for user retention and a better understanding of user diversity in informal educational communities on social media.
Keywords
1. INTRODUCTION
Informal online learning receives increased attention in educational data mining [29, 25, 41]. Learning on social media often takes place in communities; on Twitter, hashtags connect thousands of teachers [10]. As this provides meaningful learning opportunities for teacher professional development (PD), prior research argued that social media could augment traditional PD activities [19, 24, 21].
User retention has been a topic of research investigating motivational differences for online community participation [14], social presence [26], and affiliation of central users with the community [42]. Prior work showed a gradual user dropout of the #EdChat Twitter community, with mixed success in explaining user retention through user-level features [46]. Prior research identified several user-level factors that may contribute to user retention and user membership switching in online communities outside of social media [2, 27]. These features relate to Diffusion of Innovation theory [47], network centrality [8], and social presence [36]. Yet, user community retention and switching in informal educational learning communities are understudied.
This study bridges two lines of research: First, prior findings on educational Twitter communities have focused on single-community retention and engagement [46, 42]. Second, community retention studies in educational data mining have been restricted to more formal learning settings, including MOOCs [8] and higher education [1]. Studying retention and user switching in educational Twitter communities may inform learner retention in informal online learning.
We study user retention by comparing two large and structurally different educational Twitter communities: (a) a chat-based education community and (b) a more informal “teacher lounge” community. Prior work indicates that the chat-based community’s decline coincided with the lounge community’s growth [20].
This study has three main contributions: First, we provide initial evidence for identifying and developing relevant features related to community retention in educational Twitter communities. Second, we compare the importance of different features in these models. This may support subsequent intervention studies and early-warning capabilities for user retention in informal learning communities. Third, we advance existing research on multi-community membership, user switching, and retention [44, 30, 28, 45]. Compared to prior work on teacher PD on Twitter, which emphasized cognitive and interactive over social-transactional tweets to bolster community retention [9, 46], we find that social integration and users’ perceived community fit may matter most for community retention.
2. RESEARCH BACKGROUND
2.1 Diffusion of Innovation
Diffusion of Innovation (DOI) theory describes the adoption process of an idea or product by members of a social group [40]. Diffusion describes the adoption rate for innovation as mediated through communication. The framework consists of four major components: (a) innovation, (b) communication channels, (c) the social system, and (d) time.
DOI theory classifies members into “adopter types” [40]. These adopter types include innovators, early adopters, early majority, late majority, and laggards. Membership of an adopter type is determined by the time of adoption during the diffusion process and is often considered predictive of different behavior within the diffusion process [40]. Quantitative modeling suggests that generalizations of DOI theory can have sufficient predictive power [6].
DOI has been used prominently to explain and predict innovations in the context of social media [37, 35, 22]. Studying Twitter, prior research has used DOI to investigate factors mediating adoption by various social and political groups [39, 5, 4, 34]. This approach was extended to study the continued or discontinued use of Twitter after its initial adoption using a combination of DOI and the “uses and gratifications theory” [16, 14, 15]. Notably, prior work generally overlooks the complexities of competition between communities. This work addresses this gap by using DOI to investigate community switching behavior.
2.2 Multi-group Membership and Switching
Multi-community membership refers to users simultaneously engaging with two or more distinct user groups, with these communities typically situated in related domains. Multi-community membership can be synergistic and facilitate between-group connections. On Twitter, exchanges across hashtagged communities could form and reform multi-directional connections, effectively bridging communities and leveraging their resources and audiences [30]. Cross-community ties can also emerge due to users switching networks and retaining links to their old community [45].
Multi-community membership can facilitate between-group competition, affecting community growth and thriving [44]. This suggests that larger and older groups may experience difficulty growing their membership and are more vulnerable to competitive pressure. At the same time, community members identify community leaders via sociability, knowledge contribution behaviors, and structural social capital (often operationalized via betweenness centrality; [18]). Therefore, influential users play a central role in the competition between established and emerging communities.
Connections in emerging communities are based on geographic and social similarities between users. For example, the types of social ties in communities are often correlated with community age [28]. In established communities, sharing is more predicated on expertise. Moreover, prior work found user membership characteristics (e.g., engagement rate, professional role, and user account age) to relate to the sentiment in educational Twitter communities [41]. Given these systematic differences in online communities, research may exploit these variations to predict community membership. Prior work on such predictive models is scarce but indicated that models might predict community membership via social ties, user attribute homophily, and existing community memberships for a set of game-based communities [3].
2.3 The Present Study
This study investigates two large educational Twitter communities
with considerable user and time overlap regarding user
activity. Prior research on user community switching and
retention primarily focused on non-educational communities
[44, 30, 28, 45] and put limited focus on explaining the
determinants of user switching based on community- and
user-level features. Understanding the determinants of user
switching and its timing offers novel lenses into user switching
behavior and opens up the potential to intervene and retain
users in educational communities. We investigate the following
three research questions (RQs):
RQ1: How did the user base of two large educational Twitter
communities overlap over time according to the Diffusion of
Innovation model?
RQ2: How can user switching between two large educational
Twitter communities be inferred using community membership
and user-level features?
RQ3: How can the relative timing of user switches between two
large educational Twitter communities be inferred using
community membership and user-level features?
3. METHOD
3.1 Sample Description
This study uses data from a large project that examined the entire German educational Twittersphere [20]. The corresponding data download occurred between April 8 - 25, 2022, with the Twitter API 2.1. Our sample includes all tweets until the end of 2021 in Germany’s two largest educational Twitter communities: the EdChatDE and the TWLZ community.
EdChatDE is the German chapter of the American EdChat network, which holds and facilitates regular chat hours for educators. In contrast, the TWLZ (an abbreviation for “Twitterlehrerzimmer,” which translates to “Twitter teacher’s lounge”) is an umbrella community for education professionals to talk, connect, and share content across subject areas and school levels.
Notably, our data does not only include tweets that used the sampled hashtags but also all of their conversation tweets (i.e., replies to the tweets, including the respective hashtags). We removed 255\(,\)061 tweets from 170 identified bot accounts. Bot detection followed a hybrid approach based on keyword filtering in user bios and human coding as described in [20]. This led to a full study sample of 2\(,\)039\(,\)260 tweets from 104\(,\)847 unique users.
3.2 Measures
This study infers user switching and timing from user roles based on the DOI theory and other features mined from Twitter data. The code of our analyses is publicly available.1
Community membership and switching. We defined community membership as users having at least two community interactions (i.e., mentioning, quoting, replying, or retweeting another user in a post that contributed to the community). This number was determined by investigating a logistic growth model’s fit via \(RSS\) (as assumed by the DOI model). We checked the robustness of our results across one, two, and three required interactions.
Notably, the last 10% of user interactions are not counted as community interactions. This hedges against artificially prolonging users’ community membership beyond a long break through random postings long after their primary community engagement ended. We verified the robustness of our results against a 15% and 5% cutoff.
We define user switching as the point in time when users become members of one community and cease to be members of another community (Equation 1). Our definition allows for a time gap where users are members of neither community before joining the community to which they switch. \begin {equation} t_{switch[a \rightarrow b]} = max(t_{exit[a]}, t_{entry[b]}) \;\;|\;\; t_{exit[b]} > t_{exit[a]} \end {equation}
DOI user types. We classified users based on the time they joined the chat community following DOI theory [32]. Users are ranked based on how early they joined a community, with groups separated by quantiles. This results in the following variable levels: innovators (earliest 2.5%), early adopters (13.5%), early majority (34%), late majority (34%), and laggards (remaining 16%).
Social network analysis. To operationalize the social status of users within a community, we calculate a set of common centrality measures, including degree, closeness, betweenness, and eigenvector centrality on an unweighted and undirected network of user-to-user interactions [7]. Degree centrality refers to the number of nodes (i.e., other users) a user has interacted with. Closeness centrality measures the distance between a node and every other node in the network. That means well-connected users have shorter connection paths to all other users. Betweenness centrality is a measure of a node’s function as a bridge to connect other nodes. Eigenvector centrality describes the importance of a node by the sum of the centrality of the nodes it connects to. Thus, a user interacting with a central user gains importance in the network.
Teacher classification. We trained a supervised learning classifier based on a training data set of 1\(,\)000 randomly-sampled user profiles [38]. Two experienced human coders first labeled users’ Twitter bios and up to 50 sample tweets into “teachers” and “non-teachers.” Their inter-rater reliability was substantial with \(\kappa = 0.77\) [12]. Subsequently, we trained and tested multiple text-based teacher classification models using different algorithms, from which a logistic regression model emerged as the most predictive. The model was optimized with hyperparameter tuning via grid search and 10-fold cross-validation and achieved a holdout test set (\(N = 250\)) accuracy of \(AUC = 0.79\). Applied to our study sample, 10\(,\)313 users were classified as teachers, contributing 1\(,\)078\(,\)976 tweets while 94\(,\)534 non-teachers contributed 960\(,\)284 tweets.
Twitter engagement. User and social engagement variables are provided by the Twitter API, including a continuous variable on the user’s lifespan, that is, the time passed (in days) since they first joined Twitter. Also, we included two continuous variables indicating the number of followers and followings. This allows us to gauge a user’s popularity and connectedness on the platform. Lastly, we included two continuous variables describing users’ posting behavior: the average number of tweets a user posted per day and their total number of reposts (i.e., retweets and quotes). These measures indicate a user’s level of engagement and responsiveness.
3.3 Analytical Methods
RQ1 reports descriptive statistics on the size and overlap of two large educational Twitter communities. Then, we describe the descriptive overlap in DOI membership types between these two communities for active users.
RQ2 applies logistic regression models to infer user switching behavior. We employ \(AIC\)-based backward search to determine a parsimonious and interpretable user switching model [31]. We \(z\)-standardized all numeric variables to aid model coefficient interpretations. Additionally, we log-transformed network centrality measures before standardizing them, given their heavy-tailed distributions [17]. For all linear models, we verified that modeling assumptions (e.g., normal distribution of residuals, homoscedasticity, and linearity assumptions) are not violated through inspection of corresponding diagnostic plots.
RQ3 replicates the modeling procedure presented in RQ2 using the \(z\)-standardized relative timing of user switching as the dependent variable with ordinary least squares (OLS) regression models.
4. RESULTS
4.1 Community User Overlap (RQ1)
The chat community included \(N=5{,}391\) members, while the lounge community included \(N= 69{,}877\) members. \(N = 2{,}775\) users had dual membership for an average of \(M = 243\) days (\(SD\) = 273 days). The median number of active days was 1\(,\)273 for the chat community and 317 for the lounge community. We report the longitudinal development of user numbers in both communities in Figure 1. Most notably, the decline of user numbers in the chat-based community occurred shortly after the lounge community experienced exponential user growth starting in 2017.
We found that \(N = 2{,}891\) (65.98%) of chat community members switched to the Twitter lounge. The median switching time was 842 days after joining the chat-based community with an \(IRQ\) of 1\(,\)218 days, yielding a considerable variance in whether users switched and their exact time of switching. Associations between the DOI user types of both communities are displayed in Figure 2.
Figure 2 indicates that chat community laggards were often early adopters of the teacher lounge community. Notably, these users were also less often innovators in the teacher lounge community. Conversely, the late majority of the chat-based community were more often innovators and less often early adopters of the teacher lounge community. A \(\chi ^2\) independence test rejected the independence of both user classifications (\(\chi ^2\) = 320.82, \(df\) = 16, \(p\) \(<\) .001).
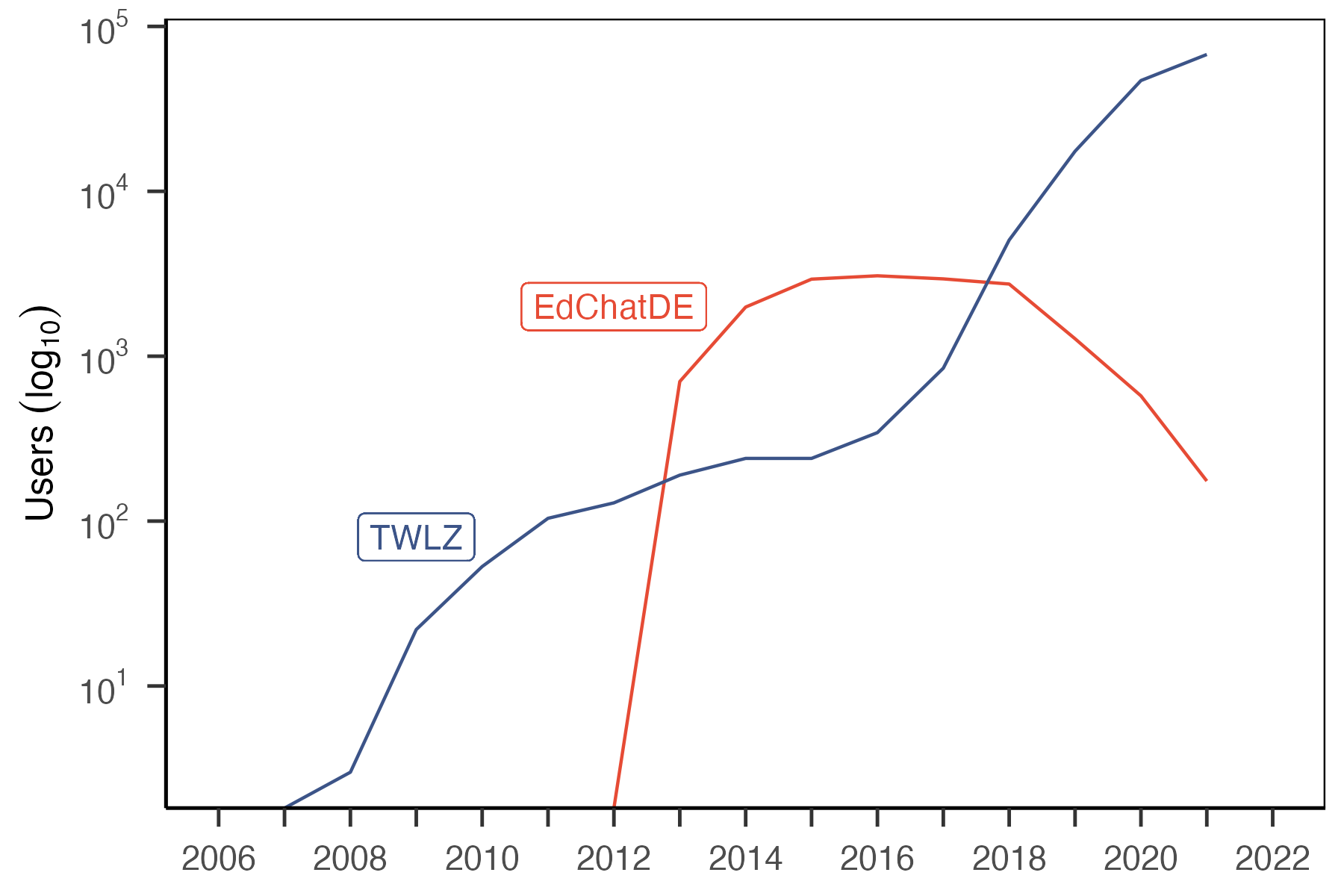
4.2 Determinants of Community Switching (RQ2)
We investigate user switching determinants from the chat community to the teacher lounge community via logistic regression (Table 1). The four main findings are as follows:
First, the later users joined the chat community, the more likely they were to switch to the teacher lounge community. Effect sizes ranged between \(OR\) = 1.10 (\(p = .863\)) for early adopters compared to innovators and \(OR\) = 296.87 (\(p < .001\)) for early adopters compared to innovators. Second, users whose Twitter account was a standard deviation older than average were around 24 times more likely to switch (\(OR = 24.49\), \(p < .001 \)). Third, teachers were more than three times as likely to switch to the teacher lounge community than non-teachers (\(OR = 3.06\), \(p < .001\)). Fourth, users with larger followings and more followed accounts were less likely to switch (\(OR = 0.76\), \(p < .001\) and \(OR = 0.60\), \(p < .001\), respectively). Fourth, among our centrality measures, degree centrality exhibited the largest effect size and was positively associated with user switching (\(OR = 2.48\), \(p < .001 \)).
4.3 Timing of Community Switching (RQ3)
Analogous to RQ2, we investigate the determinants of the relative timing of user switching using ordinary least squares regression (Table 2). The three main findings are as follows:
First, users that joined the chat community later were also more likely to join the teacher lounge community later. While early adopters joined the teacher lounge community \(\beta = 0.13\) standard deviations (approx. 50 days) later (\(p = .055\)) compared to innovators, laggards joined the teacher lounge community \(\beta = 0.42\) standard deviations (approx. 163 days) later (\(p < .001\)) compared to innovators. Second, teachers switched \(\beta = -0.29\) standard deviations (approx. 112 days) earlier (\(p < .001\)) than non-teachers. Third, degree centrality was most strongly associated with the relative timing of user switching. Per additional standard deviation in user degree centrality, users switched \(\beta = -0.37\) standard deviations (approx. 143 days) earlier (\(p < .001\)).
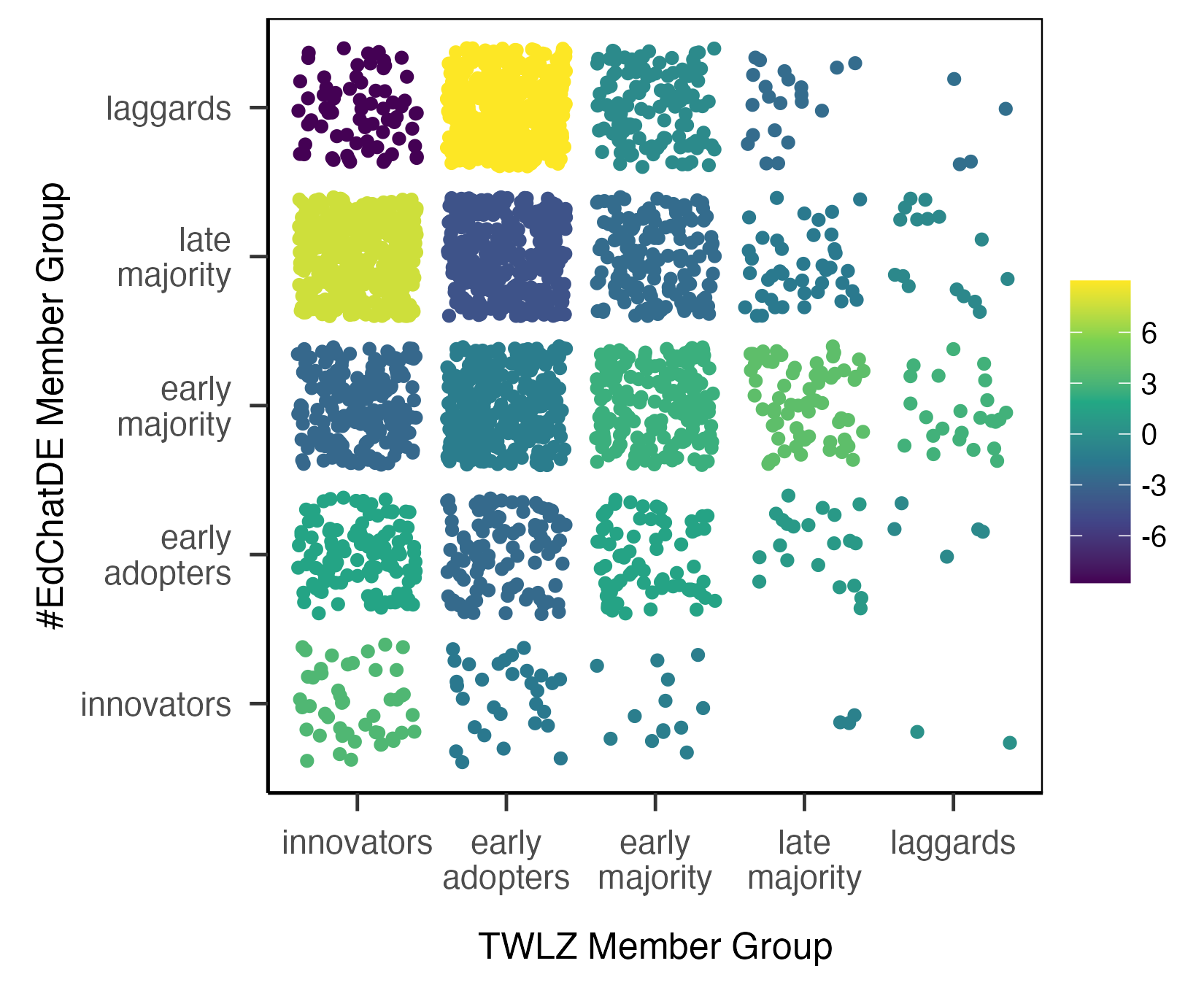
Effect | \(OR\) | \(SE\) |
---|---|---|
(Intercept) | 0.05*** | 0.51 |
Chat DOI group [vs. innovators] | ||
Early adopters | 1.10 | 0.53 |
Early majority | 9.34*** | 0.51 |
Late majority | 60.10*** | 0.53 |
Laggards | 296.87*** | 0.55 |
Teacher [vs. non-teacher] | 3.06*** | 0.18 |
Lifespan (days) | 24.49*** | 0.11 |
User following | 0.60*** | 0.09 |
User followers | 0.76*** | 0.08 |
Chat tweets per day | 0.76*** | 0.06 |
Degree Centrality | 2.48*** | 0.14 |
Closeness Centrality | 0.63*** | 0.07 |
Betweenness Centrality | 1.61*** | 0.10 |
Observations 5,391 | ||
\(R^2\) Tjur 0.785 | ||
* \(p<.05\), ** \(p<.01\), *** \(p<.001\) |
User classification features had among the largest effect sizes. Figure 3 illustrates interactions between DOI and teacher user classifications to further examine associations of DOI types across different user types. Notably, teachers consistently switched communities across all DOI user types earlier than non-teachers. However, the difference between teachers and non-teachers diminished the later users switched. In particular, the smallest difference in medians between teachers and non-teachers was for the late majority (0.12 \(SD\); approx. 48 days) and laggards (0.43 \(SD\); approx. 166 days) of the chat-based community.
Effect | \(\beta \) | \(SE\) |
---|---|---|
(Intercept) | -0.01 | 0.11 |
Chat DOI group [vs. innovators] | ||
Early adopters | 0.14 | 0.11 |
Early majority | 0.18 | 0.11 |
Late majority | -0.06 | 0.12 |
Laggards | 0.43** | 0.13 |
Teacher [vs. non-teacher] | -0.29*** | 0.04 |
Lifespan (days) | -0.08** | 0.03 |
User following | 0.04* | 0.02 |
User reposts | -0.04* | 0.02 |
Degree Centrality | -0.37*** | 0.04 |
Closeness Centrality | -0.17*** | 0.04 |
Betweenness Centrality | 0.16*** | 0.03 |
Eigenvector Centrality | 0.05 | 0.04 |
Observations 2,693 | ||
\(R^2\) / Adjusted \(R^2\) 0.145 / 0.141 | ||
* \(p<.05\), ** \(p<.01\), *** \(p<.001\) |
5. DISCUSSION
This study examines user switching determinants between two large education-related Twitter communities through a DOI theory lens. While prior studies focused on the growth and retention in single communities [16, 14, 15], less attention has been given to user characteristics explaining community switching. Our three main findings are as follows:
First, community switching was more likely the longer users were active on Twitter and the later they joined the community from which they switched. This is important as prior work focused on increasing cognitive and interactive tweets over social-transactional tweets to increase retention in PD communities [9, 46]. However, our findings suggest that social integration into the community matters for community retention. Users that joined a community late may have more challenges in making connections or building a reputation in the community. Therefore, they might be more likely to switch communities. Alternatively, the entry barrier may feel higher for newcomers in more established communities, given that sharing in older communities tends to be predicated on expertise rather than social and geographic similarity [28]. Notably, we found the relative community joining time measures to have large effect sizes encouraging future research to use relative community join times as measures for understanding informal learning communities.
Second, teachers were around three times more likely to switch communities and switched around 112 days earlier compared to non-teachers. Given that the community to which users switched in our sample is an “Twitter teacher lounge,” these observed effects may relate to an increased perceived fit for teachers regarding the target community [11]. This interpretation aligns with findings from marketing research investigating customer adoption of products moderated by self-identity [13, 43]. Therefore, communities may improve user adoption by explicitly addressing their target audiences. Future research may investigate more features of perceived role fit to infer educational community retention.
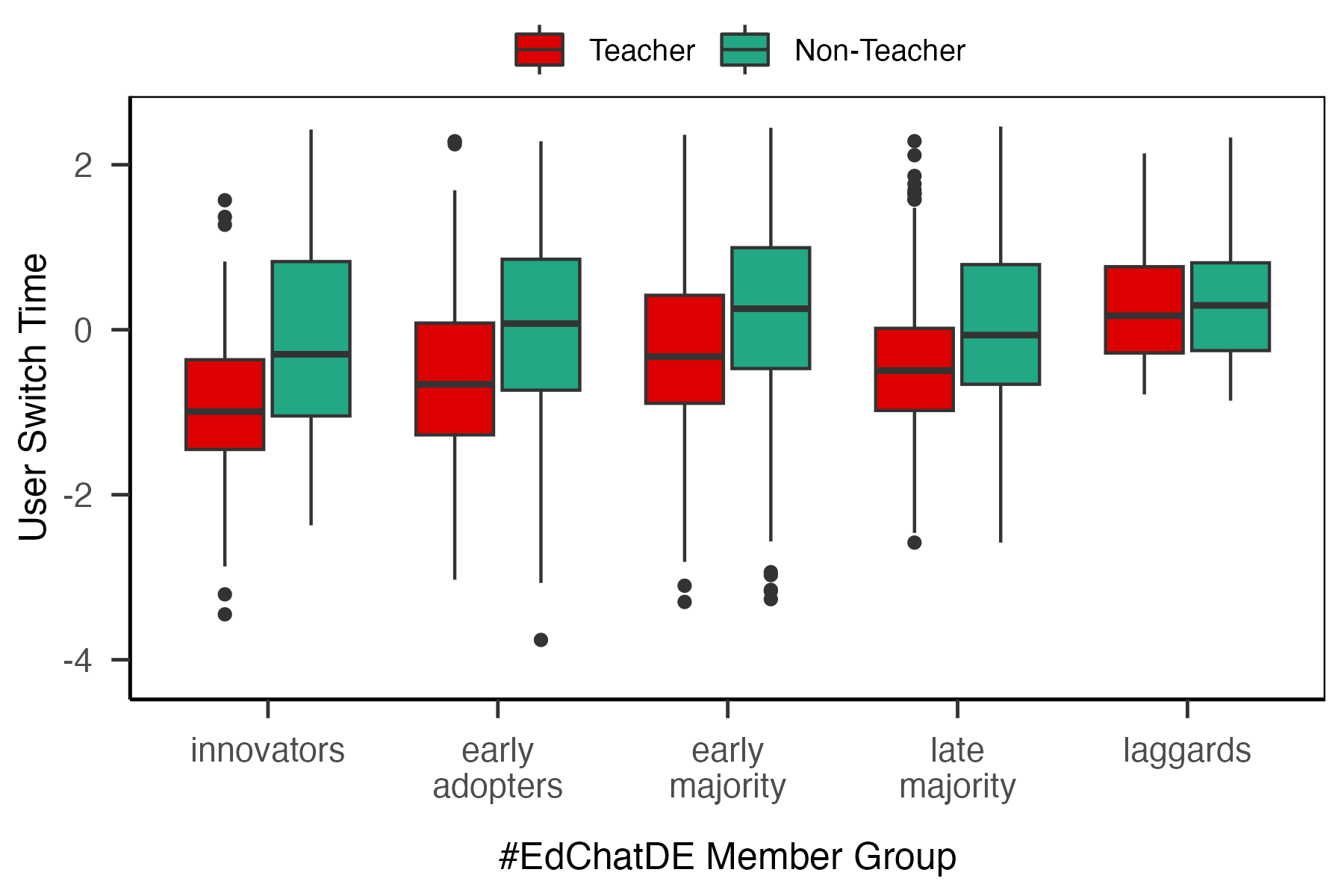
Third, central community members were more likely to switch communities. This finding extends prior work suggesting that interactions with highly influential users positively relate to community retention [42] and prolonged engagement in blogs [33]. A potential interpretation is that highly central users may have a stronger tendency to adopt and seek novel participation opportunities in educational Twitter, irrespective of their integration into the community. Alternatively, the decline of the chat-based community in our data set may be predicated on highly influential users, being the community backbones, leaving the community. Comparing both explanations in future research may improve community intervention efforts, for example, by targeting highly central users in the network to continue their engagement and boost community health and longevity.
5.1 Limitations and Future Work
This study only examined two Twitter communities. Specific events, such as the COVID-19 pandemic, may have influenced community growth, during which the teacher lounge community experienced large growth [23]. In addition, our data are correlational and do not allow for causal claims. However, future work may leverage longitudinal or hierarchical modeling with repeated user engagement measurements to better understand the strong association of DOI groups with user switching. Similarly, future work may explore user-level differences in device usage (e.g., desktop vs. mobile use) or create an early warning system flagging declining engagement of central users.
Taken together, our findings can provide important insights for stakeholders initiating, studying, and orchestrating informal PD spaces on Twitter and inform research on informal learning in digital spaces more broadly.
6. REFERENCES
- F. Agrusti, G. Bonavolontà, and M. Mezzini. University dropout prediction through educational data mining techniques: A systematic review. Journal of E-Learning and Knowledge Society, 15(3):161–182, 2019.
- T. Althoff and J. Leskovec. Donor retention in online crowdfunding communities: A case study of donorschoose. org. In Proceedings of the 24th International Conference on World Wide Web, pages 34–44, 2015.
- H. Alvari, K. Lakkaraju, G. Sukthankar, and J. Whetzel. Predicting guild membership in massively multiplayer online games. In International Conference on Social Computing, Behavioral-Cultural Modeling, and Prediction, pages 215–222. Springer, 2014.
- M. Anderson, K. Lewis, and O. Dedehayir. Diffusion of innovation in the public sector: Twitter adoption by municipal police departments in the us. In 2015 Portland International Conference on Management of Engineering and Technology (PICMET), pages 2453–2464. IEEE, 2015.
- M. M. Archibald and A. M. Clark. Twitter and nursing research: how diffusion of innovation theory can help uptake. Journal of Advanced Nursing, 70(3):e3–e5, 2014.
- F. M. Bass. A new product growth for model consumer durables. Management Science, 15(5):215–227, 1969.
- F. Bloch, M. O. Jackson, and P. Tebaldi. Centrality Measures in Networks. (arXiv:1608.05845), 2021.
- A. Bozkurt. Surfing on three waves of MOOCs: An examination and snapshot of research in massive open online courses. Open Praxis, 13(3):296–311, 2021.
- J. Carpenter and D. Krutka. Engagement through microblogging: Educator professional development via Twitter. Professional Development in Education, 41(4):707–728, 2015.
- J. P. Carpenter and S. A. Morrison. Enhancing teacher education… with Twitter? Phi Delta Kappan, 100(1):25–28, 2018.
- S. M. Chan-Olmsted, M. Cho, and S. Lee. User perceptions of social media: A comparative study of perceived characteristics and user profiles by social media. Online Journal of Communication and Media Technologies, 3(4):149–178, 2013.
- J. Cohen. A coefficient of agreement for nominal scales. Educational and Psychological Measurement, 20(1):37–46, 1960.
- I. Confente, D. Scarpi, and I. Russo. Marketing a new generation of bio-plastics products for a circular economy: The role of green self-identity, self-congruity, and perceived value. Journal of Business Research, 112:431–439, 2020.
- K. Constantinos and Y. Coursaris. Understanding Twitter’s adoption and use continuance: The synergy between uses and gratifications and diffusion of innovations. SIGHCI 2010 Proceedings. Paper, 3, 2010.
- C. K. Coursaris, W. Van Osch, J. Sung, and Y. Yun. Disentangling Twitter’s adoption and use (dis) continuance: A theoretical and empirical amalgamation of uses and gratifications and diffusion of innovations. AIS Transactions on Human-Computer Interaction, 5(1):57–83, 2013.
- C. K. Coursaris, Y. Yun, and J. Sung. Twitter Users vs. Quitters: a Uses and Gratifications and Diffusion of Innovations approach in understanding the role of mobility in microblogging. In 2010 Ninth International Conference on Mobile Business and 2010 Ninth Global Mobility Roundtable (ICMB-GMR), pages 481–486. IEEE, 2010.
- K. Dasaratha. Distributions of centrality on networks. Games and Economic Behavior, 122:1–27, 2020.
- S. Faraj, S. Kudaravalli, and M. Wasko. Leading collaboration in online communities. MIS quarterly, 39(2):393–412, 2015.
- C. Fischer, B. Fishman, and S. Y. Schoenebeck. New contexts for professional learning: Analyzing high school science teachers’ engagement on Twitter. AERA Open, 5(4):1–20, 2019.
- C. Fischer, L. Klein, C. Borchers, and F. Morina. Mapping the landscape of educational Twitter use in Germany: Informal teacher learning in online communities of practice. OSF Preprints, 2023.
- C. Fischer, Y. Omarchevska, T. Fütterer, and J. Rosenberg. How do teachers collaborate in informal professional learning activities? An epistemic network analysis. OSF Preprints, 2022.
- O. Folorunso, R. O. Vincent, A. F. Adekoya, and A. O. Ogunde. Diffusion of innovation in social networking sites among university students. International Journal of Computer Science and Security, 4(3):361–372, 2010.
- T. Fütterer, E. Hoch, K. Stürmer, A. Lachner, C. Fischer, and K. Scheiter. Was bewegt Lehrpersonen während der Schulschließungen? Eine Analyse der Kommunikation im Twitter-Lehrerzimmer über Chancen und Herausforderungen digitalen Unterrichts. Zeitschrift für Erziehungswissenschaft, 24(2):443–477, 2021.
- C. Greenhow, S. M. Galvin, D. L. Brandon, and E. Askari. A decade of research on K–12 teaching and teacher learning with social media: Insights on the state of the field. Teachers College Record, 122(6):1–72, 2020.
- A. Gruzd, D. Paulin, and C. Haythornthwaite. Analyzing social media and learning through content and social network analysis: A faceted methodological approach. Journal of Learning Analytics, 3(3):46–71, 2016.
- S. Han, J. Min, and H. Lee. Antecedents of social presence and gratification of social connection needs in SNS: a study of Twitter users and their mobile and non-mobile usage. International Journal of Information Management, 35(4):459–471, 2015.
- K. S. Hone and G. R. El Said. Exploring the factors affecting MOOC retention: A survey study. Computers & Education, 98:157–168, 2016.
- E. H. Hwang, P. V. Singh, and L. Argote. Knowledge sharing in online communities: Learning to cross geographic and hierarchical boundaries. Organization Science, 26(6):1593–1611, 2015.
- S. Joksimović, V. Kovanović, J. Jovanović, A. Zouaq, D. Gašević, and M. Hatala. What do cMOOC participants talk about in social media? a topic analysis of discourse in a cMOOC. In Proceedings of the Fifth International Conference on Learning Analytics and Knowledge, pages 156–165, 2015.
- J. Jones. Switching in Twitter’s hashtagged exchanges. Journal of Business and Technical Communication, 28(1):83–108, 2014.
- A. Jović, K. Brkić, and N. Bogunović. A review of feature selection methods with applications. In 2015 38th International Convention on Information and Communication Technology, Electronics and Microelectronics (MIPRO), pages 1200–1205. IEEE, 2015.
- J. Kaminski. Diffusion of innovation theory. Canadian Journal of Nursing Informatics, 6(2):1–6, 2011.
- I. Kayes and J. Chakareski. Retention in online blogging: a case study of the blogster community. IEEE Transactions on Computational Social Systems, 2(1):1–14, 2015.
- C. Kim and S. Lee. Innovation vs. normalization: Politicians’ Twitter use at the early majority stage of its diffusion in the Korean assembly. The Social Science Journal, pages 1–13, 2020.
- A. Leerapong. Applying diffusion of innovation in online purchase intention through social network: A focus group study of Facebook in Thailand. Information Management and Business Review, 5(3):144–154, 2013.
- S. Y. Liu, J. Gomez, and C.-J. Yen. Community college online course retention and final grade: Predictability of social presence. Journal of Interactive Online Learning, 8(2), 2009.
- L. Ma, C. S. Lee, and D. H.-L. Goh. Understanding news sharing in social media: An explanation from the diffusion of innovations theory. Online Information Review, 38(5):598–615, 2014.
- M. Pennacchiotti and A.-M. Popescu. A Machine Learning Approach to Twitter User Classification. Proceedings of the International AAAI Conference on Web and Social Media, 5(1):281–288, 2011.
- R. D. Peterson. To tweet or not to tweet: Exploring the determinants of early adoption of Twitter by House members in the 111th Congress. The Social Science Journal, 49(4):430–438, 2012.
- E. M. Rogers, A. Singhal, and M. M. Quinlan. Diffusion of innovations. In An Integrated Approach to Communication Theory and Research, pages 432–448. Routledge, 2014.
- J. M. Rosenberg, C. Borchers, E. B. Dyer, D. Anderson, and C. Fischer. Understanding public sentiment about educational reforms: The next generation science standards on Twitter. AERA open, 7:1–17, 2021.
- J. M. Rosenberg, J. W. Reid, E. B. Dyer, M. J. Koehler, C. Fischer, and T. J. McKenna. Idle chatter or compelling conversation? the potential of the social media-based #NGSSchat network for supporting science education reform efforts. Journal of Research in Science Teaching, 57(9):1322–1355, 2020.
- A. P. Schouten, L. Janssen, and M. Verspaget. Celebrity vs. influencer endorsements in advertising: the role of identification, credibility, and product-endorser fit. International journal of advertising, 39(2):258–281, 2020.
- X. Wang, B. S. Butler, and Y. Ren. The impact of membership overlap on growth: An ecological competition view of online groups. Organization Science, 24(2):414–431, 2013.
- Y. Wei, W. Zhang, S. Yang, and X. Chen. Online social network with communities: Evolvement of within-and cross-community ties. Available at SSRN 3420525, 2021.
- W. Xing and F. Gao. Exploring the relationship between online discourse and commitment in Twitter professional learning communities. Computers & Education, 126:388–398, 2018.
- M. Zhu. Analysis of technology innovation diffusion model and diffusion characteristics on educational technology: Case study of MOOC. In Proceedings of the 2022 5th International Conference on Mathematics and Statistics, pages 47–51, 2022.
1github.com/conradborchers/community-switch-edm23
© 2023 Copyright is held by the author(s). This work is distributed under the Creative Commons Attribution-NonCommercial-NoDerivatives 4.0 International (CC BY-NC-ND 4.0) license.